Animating NEXRAD Level II Data#
Overview#
Within this notebook, we will cover:
Exploring the “guts” of a NEXRAD radar file
Animating a sequence of AWS-served NEXRAD Level 2 Radar scans
Multi-hour plots
Prerequisites#
Concepts |
Importance |
Notes |
---|---|---|
Required |
Projections and Features |
|
Required |
Basic plotting |
|
Required |
IO/Visualization |
Time to learn: 20 minutes
Imports#
import pyart
import fsspec
from metpy.plots import USCOUNTIES, ctables
import matplotlib.pyplot as plt
import cartopy.crs as ccrs
import cartopy.feature as cfeature
import warnings
from datetime import datetime as dt
from datetime import timedelta
import pandas as pd
import matplotlib
matplotlib.rcParams['animation.html'] = 'html5'
from matplotlib.animation import ArtistAnimation
warnings.filterwarnings("ignore")
## You are using the Python ARM Radar Toolkit (Py-ART), an open source
## library for working with weather radar data. Py-ART is partly
## supported by the U.S. Department of Energy as part of the Atmospheric
## Radiation Measurement (ARM) Climate Research Facility, an Office of
## Science user facility.
##
## If you use this software to prepare a publication, please cite:
##
## JJ Helmus and SM Collis, JORS 2016, doi: 10.5334/jors.119
Select the time and NEXRAD site#
start_time = dt(2025,4,26,17)
start_time_str = dt.strftime(start_time, format="%Y%m%d%H")
site = 'KENX'
Select number of hours. 1 hour is strongly recommended!#
nHours = 4
Point to the NOAA NEXRAD Level 2 S3 Bucket on AWS
fs = fsspec.filesystem("s3", anon=True)
fileList = []
for n in range(0, nHours):
datTime = start_time + timedelta(hours = n)
year = dt.strftime(datTime,format="%Y")
month = dt.strftime(datTime,format="%m")
day = dt.strftime(datTime,format="%d")
hour = dt.strftime(datTime,format="%H")
timeStr = f'{year}{month}{day}{hour}'
# Depending on the year, the radar files will have different naming conventions.
pattern1 = f's3://noaa-nexrad-level2/{year}/{month}/{day}/{site}/{site}{year}{month}{day}_{hour}*V06'
pattern2 = f's3://noaa-nexrad-level2/{year}/{month}/{day}/{site}/{site}{year}{month}{day}_{hour}*V*.gz'
pattern3 = f's3://noaa-nexrad-level2/{year}/{month}/{day}/{site}/{site}{year}{month}{day}_{hour}*.gz'
files = sorted(fs.glob(pattern1))
if (len(files) == 0):
files = sorted(fs.glob(pattern2))
if (len(files) == 0):
files = sorted(fs.glob(pattern3))
fileList.extend(files)
If we have an empty list, either there are no files available for that site/date, or the file name does not match any of the patterns above.
if (len(fileList) == 0):
print ("There are no files found for this date and location. Either try a different date/site, \
or browse the NEXRAD2 archive to see if the file name uses a different pattern.")
else:
print (fileList)
['noaa-nexrad-level2/2025/04/26/KENX/KENX20250426_170535_V06', 'noaa-nexrad-level2/2025/04/26/KENX/KENX20250426_171110_V06', 'noaa-nexrad-level2/2025/04/26/KENX/KENX20250426_171646_V06', 'noaa-nexrad-level2/2025/04/26/KENX/KENX20250426_172207_V06', 'noaa-nexrad-level2/2025/04/26/KENX/KENX20250426_172728_V06', 'noaa-nexrad-level2/2025/04/26/KENX/KENX20250426_173233_V06', 'noaa-nexrad-level2/2025/04/26/KENX/KENX20250426_173739_V06', 'noaa-nexrad-level2/2025/04/26/KENX/KENX20250426_174231_V06', 'noaa-nexrad-level2/2025/04/26/KENX/KENX20250426_174723_V06', 'noaa-nexrad-level2/2025/04/26/KENX/KENX20250426_175216_V06', 'noaa-nexrad-level2/2025/04/26/KENX/KENX20250426_175652_V06', 'noaa-nexrad-level2/2025/04/26/KENX/KENX20250426_180129_V06', 'noaa-nexrad-level2/2025/04/26/KENX/KENX20250426_180607_V06', 'noaa-nexrad-level2/2025/04/26/KENX/KENX20250426_181044_V06', 'noaa-nexrad-level2/2025/04/26/KENX/KENX20250426_181522_V06', 'noaa-nexrad-level2/2025/04/26/KENX/KENX20250426_181959_V06', 'noaa-nexrad-level2/2025/04/26/KENX/KENX20250426_182505_V06', 'noaa-nexrad-level2/2025/04/26/KENX/KENX20250426_182844_V06', 'noaa-nexrad-level2/2025/04/26/KENX/KENX20250426_183301_V06', 'noaa-nexrad-level2/2025/04/26/KENX/KENX20250426_183718_V06', 'noaa-nexrad-level2/2025/04/26/KENX/KENX20250426_184202_V06', 'noaa-nexrad-level2/2025/04/26/KENX/KENX20250426_184619_V06', 'noaa-nexrad-level2/2025/04/26/KENX/KENX20250426_185051_V06', 'noaa-nexrad-level2/2025/04/26/KENX/KENX20250426_185528_V06', 'noaa-nexrad-level2/2025/04/26/KENX/KENX20250426_190013_V06', 'noaa-nexrad-level2/2025/04/26/KENX/KENX20250426_190458_V06', 'noaa-nexrad-level2/2025/04/26/KENX/KENX20250426_190936_V06', 'noaa-nexrad-level2/2025/04/26/KENX/KENX20250426_191421_V06', 'noaa-nexrad-level2/2025/04/26/KENX/KENX20250426_191906_V06', 'noaa-nexrad-level2/2025/04/26/KENX/KENX20250426_192350_V06', 'noaa-nexrad-level2/2025/04/26/KENX/KENX20250426_192828_V06', 'noaa-nexrad-level2/2025/04/26/KENX/KENX20250426_193301_V06', 'noaa-nexrad-level2/2025/04/26/KENX/KENX20250426_193746_V06', 'noaa-nexrad-level2/2025/04/26/KENX/KENX20250426_194219_V06', 'noaa-nexrad-level2/2025/04/26/KENX/KENX20250426_194656_V06', 'noaa-nexrad-level2/2025/04/26/KENX/KENX20250426_195134_V06', 'noaa-nexrad-level2/2025/04/26/KENX/KENX20250426_195612_V06', 'noaa-nexrad-level2/2025/04/26/KENX/KENX20250426_200050_V06', 'noaa-nexrad-level2/2025/04/26/KENX/KENX20250426_200528_V06', 'noaa-nexrad-level2/2025/04/26/KENX/KENX20250426_200946_V06', 'noaa-nexrad-level2/2025/04/26/KENX/KENX20250426_201404_V06', 'noaa-nexrad-level2/2025/04/26/KENX/KENX20250426_201823_V06', 'noaa-nexrad-level2/2025/04/26/KENX/KENX20250426_202242_V06', 'noaa-nexrad-level2/2025/04/26/KENX/KENX20250426_202700_V06', 'noaa-nexrad-level2/2025/04/26/KENX/KENX20250426_203119_V06', 'noaa-nexrad-level2/2025/04/26/KENX/KENX20250426_203537_V06', 'noaa-nexrad-level2/2025/04/26/KENX/KENX20250426_203956_V06', 'noaa-nexrad-level2/2025/04/26/KENX/KENX20250426_204415_V06', 'noaa-nexrad-level2/2025/04/26/KENX/KENX20250426_204820_V06', 'noaa-nexrad-level2/2025/04/26/KENX/KENX20250426_205211_V06', 'noaa-nexrad-level2/2025/04/26/KENX/KENX20250426_205550_V06', 'noaa-nexrad-level2/2025/04/26/KENX/KENX20250426_205929_V06']
Read the Data into PyART#
Read in the first radar file in the group and list the available fields.
radar = pyart.io.read_nexrad_archive(f's3://{fileList[0]}')
list(radar.fields)
['reflectivity',
'spectrum_width',
'cross_correlation_ratio',
'velocity',
'clutter_filter_power_removed',
'differential_phase',
'differential_reflectivity']
The radar
object has a lot of useful data … and metadata! One way to look at the attributes of any Python object is to use the vars
function. It returns a Python dictionary
; each dictionary key
has an associated value … which might be a single value … a list of values … another dictionary … and so on.
radarDict = vars(radar)
radarDict
{'time': {'units': 'seconds since 2025-04-26T17:05:35Z',
'standard_name': 'time',
'long_name': 'time_in_seconds_since_volume_start',
'calendar': 'gregorian',
'comment': 'Coordinate variable for time. Time at the center of each ray, in fractional seconds since the global variable time_coverage_start',
'data': array([ 0.516, 0.556, 0.599, ..., 327.1 , 327.136, 327.174])},
'range': {'units': 'meters',
'standard_name': 'projection_range_coordinate',
'long_name': 'range_to_measurement_volume',
'axis': 'radial_range_coordinate',
'spacing_is_constant': 'true',
'comment': 'Coordinate variable for range. Range to center of each bin.',
'data': array([ 2125., 2375., 2625., ..., 459375., 459625., 459875.],
dtype=float32),
'meters_to_center_of_first_gate': 2125.0,
'meters_between_gates': 250.0},
'fields': {'reflectivity': {'units': 'dBZ',
'standard_name': 'equivalent_reflectivity_factor',
'long_name': 'Reflectivity',
'valid_max': 94.5,
'valid_min': -32.0,
'coordinates': 'elevation azimuth range',
'_FillValue': -9999.0,
'data': masked_array(
data=[[-22.0, --, -18.0, ..., --, --, --],
[23.0, 15.5, 10.5, ..., --, --, --],
[23.0, 20.5, 10.5, ..., --, --, --],
...,
[28.0, 29.5, 43.0, ..., --, --, --],
[20.5, 21.5, 44.0, ..., --, --, --],
[22.5, 24.5, 42.0, ..., --, --, --]],
mask=[[False, True, False, ..., True, True, True],
[False, False, False, ..., True, True, True],
[False, False, False, ..., True, True, True],
...,
[False, False, False, ..., True, True, True],
[False, False, False, ..., True, True, True],
[False, False, False, ..., True, True, True]],
fill_value=np.float64(1e+20),
dtype=float32)},
'spectrum_width': {'units': 'meters_per_second',
'standard_name': 'doppler_spectrum_width',
'long_name': 'Spectrum Width',
'valid_max': 63.0,
'valid_min': -63.5,
'coordinates': 'elevation azimuth range',
'_FillValue': -9999.0,
'data': masked_array(
data=[[--, --, --, ..., --, --, --],
[--, --, --, ..., --, --, --],
[--, --, --, ..., --, --, --],
...,
[0.0, 0.0, 0.5, ..., --, --, --],
[1.0, 3.0, 0.0, ..., --, --, --],
[1.0, 1.5, 0.0, ..., --, --, --]],
mask=[[ True, True, True, ..., True, True, True],
[ True, True, True, ..., True, True, True],
[ True, True, True, ..., True, True, True],
...,
[False, False, False, ..., True, True, True],
[False, False, False, ..., True, True, True],
[False, False, False, ..., True, True, True]],
fill_value=np.float64(1e+20),
dtype=float32)},
'cross_correlation_ratio': {'units': 'ratio',
'standard_name': 'cross_correlation_ratio_hv',
'long_name': 'Cross correlation_ratio (RHOHV)',
'valid_max': 1.0,
'valid_min': 0.0,
'coordinates': 'elevation azimuth range',
'_FillValue': -9999.0,
'data': masked_array(
data=[[0.6150000095367432, --, 0.9883333444595337, ..., --, --, --],
[0.8216666579246521, 0.9683333039283752, 0.9950000047683716, ...,
--, --, --],
[0.8116666674613953, 0.8883333206176758, 0.9549999833106995, ...,
--, --, --],
...,
[0.9950000047683716, 0.9983333349227905, 0.9950000047683716, ...,
--, --, --],
[0.9816666841506958, 0.9983333349227905, 0.9950000047683716, ...,
--, --, --],
[0.9983333349227905, 0.9983333349227905, 0.9950000047683716, ...,
--, --, --]],
mask=[[False, True, False, ..., True, True, True],
[False, False, False, ..., True, True, True],
[False, False, False, ..., True, True, True],
...,
[False, False, False, ..., True, True, True],
[False, False, False, ..., True, True, True],
[False, False, False, ..., True, True, True]],
fill_value=np.float64(1e+20),
dtype=float32)},
'velocity': {'units': 'meters_per_second',
'standard_name': 'radial_velocity_of_scatterers_away_from_instrument',
'long_name': 'Mean doppler Velocity',
'valid_max': 95.0,
'valid_min': -95.0,
'coordinates': 'elevation azimuth range',
'_FillValue': -9999.0,
'data': masked_array(
data=[[--, --, --, ..., --, --, --],
[--, --, --, ..., --, --, --],
[--, --, --, ..., --, --, --],
...,
[5.0, 5.0, 3.5, ..., --, --, --],
[4.5, 5.0, 3.0, ..., --, --, --],
[5.0, 4.5, 3.0, ..., --, --, --]],
mask=[[ True, True, True, ..., True, True, True],
[ True, True, True, ..., True, True, True],
[ True, True, True, ..., True, True, True],
...,
[False, False, False, ..., True, True, True],
[False, False, False, ..., True, True, True],
[False, False, False, ..., True, True, True]],
fill_value=np.float64(1e+20),
dtype=float32)},
'clutter_filter_power_removed': {'units': 'dB',
'long_name': 'Clutter filter power removed',
'standard_name': 'clutter_filter_power_removed',
'valid_max': 73.0,
'valid_min': 0.0,
'coordinates': 'elevation azimuth range',
'_FillValue': -9999.0,
'data': masked_array(
data=[[43.0, 55.0, 26.0, ..., --, --, --],
[--, -6.0, -6.0, ..., --, --, --],
[--, --, --, ..., --, --, --],
...,
[--, -6.0, --, ..., --, --, --],
[-6.0, -6.0, --, ..., --, --, --],
[0.0, -6.0, --, ..., --, --, --]],
mask=[[False, False, False, ..., True, True, True],
[ True, False, False, ..., True, True, True],
[ True, True, True, ..., True, True, True],
...,
[ True, False, True, ..., True, True, True],
[False, False, True, ..., True, True, True],
[False, False, True, ..., True, True, True]],
fill_value=np.float64(1e+20),
dtype=float32)},
'differential_phase': {'units': 'degrees',
'standard_name': 'differential_phase_hv',
'long_name': 'differential_phase_hv',
'valid_max': 360.0,
'valid_min': 0.0,
'coordinates': 'elevation azimuth range',
'_FillValue': -9999.0,
'data': masked_array(
data=[[230.95094299316406, --, 61.35185241699219, ..., --, --, --],
[66.2882080078125, 58.88367462158203, 45.4849967956543, ..., --,
--, --],
[114.59397888183594, 68.40379333496094, 63.46743392944336, ...,
--, --, --],
...,
[62.76224136352539, 62.76224136352539, 64.52522277832031, ...,
--, --, --],
[69.46157836914062, 71.92976379394531, 64.1726303100586, ..., --,
--, --],
[59.23627471923828, 59.588871002197266, 66.2882080078125, ...,
--, --, --]],
mask=[[False, True, False, ..., True, True, True],
[False, False, False, ..., True, True, True],
[False, False, False, ..., True, True, True],
...,
[False, False, False, ..., True, True, True],
[False, False, False, ..., True, True, True],
[False, False, False, ..., True, True, True]],
fill_value=np.float64(1e+20),
dtype=float32)},
'differential_reflectivity': {'units': 'dB',
'standard_name': 'log_differential_reflectivity_hv',
'long_name': 'log_differential_reflectivity_hv',
'valid_max': 7.9375,
'valid_min': -7.875,
'coordinates': 'elevation azimuth range',
'_FillValue': -9999.0,
'data': masked_array(
data=[[4.0, --, 0.09375, ..., --, --, --],
[6.375, 1.09375, -0.5625, ..., --, --, --],
[0.25, 3.78125, -1.75, ..., --, --, --],
...,
[0.1875, 0.1875, 0.53125, ..., --, --, --],
[-0.3125, -0.6875, 0.53125, ..., --, --, --],
[-0.4375, -0.46875, 0.84375, ..., --, --, --]],
mask=[[False, True, False, ..., True, True, True],
[False, False, False, ..., True, True, True],
[False, False, False, ..., True, True, True],
...,
[False, False, False, ..., True, True, True],
[False, False, False, ..., True, True, True],
[False, False, False, ..., True, True, True]],
fill_value=np.float64(1e+20),
dtype=float32)}},
'metadata': {'Conventions': 'CF/Radial instrument_parameters',
'version': '1.3',
'title': '',
'institution': '',
'references': '',
'source': '',
'history': '',
'comment': '',
'instrument_name': 'KENX',
'original_container': 'NEXRAD Level II',
'vcp_pattern': 215},
'scan_type': 'ppi',
'latitude': {'long_name': 'Latitude',
'standard_name': 'Latitude',
'units': 'degrees_north',
'data': array([42.58655548])},
'longitude': {'long_name': 'Longitude',
'standard_name': 'Longitude',
'units': 'degrees_east',
'data': array([-74.06408691])},
'altitude': {'long_name': 'Altitude',
'standard_name': 'Altitude',
'units': 'meters',
'positive': 'up',
'data': array([589.])},
'altitude_agl': None,
'sweep_number': {'units': 'count',
'standard_name': 'sweep_number',
'long_name': 'Sweep number',
'data': array([ 0, 1, 2, 3, 4, 5, 6, 7, 8, 9, 10, 11, 12, 13, 14, 15],
dtype=int32)},
'sweep_mode': {'units': 'unitless',
'standard_name': 'sweep_mode',
'long_name': 'Sweep mode',
'comment': 'Options are: "sector", "coplane", "rhi", "vertical_pointing", "idle", "azimuth_surveillance", "elevation_surveillance", "sunscan", "pointing", "manual_ppi", "manual_rhi"',
'data': array([b'azimuth_surveillance', b'azimuth_surveillance',
b'azimuth_surveillance', b'azimuth_surveillance',
b'azimuth_surveillance', b'azimuth_surveillance',
b'azimuth_surveillance', b'azimuth_surveillance',
b'azimuth_surveillance', b'azimuth_surveillance',
b'azimuth_surveillance', b'azimuth_surveillance',
b'azimuth_surveillance', b'azimuth_surveillance',
b'azimuth_surveillance', b'azimuth_surveillance'], dtype='|S20')},
'fixed_angle': {'long_name': 'Target angle for sweep',
'units': 'degrees',
'standard_name': 'target_fixed_angle',
'data': array([ 0.48339844, 0.48339844, 0.87890625, 0.87890625, 1.3183594 ,
1.3183594 , 1.8017578 , 2.4169922 , 3.1201172 , 3.9990234 ,
5.0976562 , 6.4160156 , 7.998047 , 10.019531 , 11.99707 ,
14.018555 ], dtype=float32)},
'sweep_start_ray_index': {'long_name': 'Index of first ray in sweep, 0-based',
'units': 'count',
'data': array([ 0, 720, 1440, 2160, 2880, 3600, 4320, 4680, 5040, 5400, 5760,
6120, 6480, 6840, 7200, 7560], dtype=int32)},
'sweep_end_ray_index': {'long_name': 'Index of last ray in sweep, 0-based',
'units': 'count',
'data': array([ 719, 1439, 2159, 2879, 3599, 4319, 4679, 5039, 5399, 5759, 6119,
6479, 6839, 7199, 7559, 7919], dtype=int32)},
'target_scan_rate': None,
'rays_are_indexed': None,
'ray_angle_res': None,
'azimuth': {'units': 'degrees',
'standard_name': 'beam_azimuth_angle',
'long_name': 'azimuth_angle_from_true_north',
'axis': 'radial_azimuth_coordinate',
'comment': 'Azimuth of antenna relative to true north',
'data': array([167.25311279, 167.74200439, 168.24737549, ..., 111.50024414,
112.49176025, 113.49975586])},
'elevation': {'units': 'degrees',
'standard_name': 'beam_elevation_angle',
'long_name': 'elevation_angle_from_horizontal_plane',
'axis': 'radial_elevation_coordinate',
'comment': 'Elevation of antenna relative to the horizontal plane',
'data': array([ 0.64819336, 0.6069946 , 0.5657959 , ..., 13.974609 ,
13.974609 , 13.974609 ], dtype=float32)},
'scan_rate': None,
'antenna_transition': None,
'rotation': None,
'tilt': None,
'roll': None,
'drift': None,
'heading': None,
'pitch': None,
'georefs_applied': None,
'instrument_parameters': {'unambiguous_range': {'units': 'meters',
'comments': 'Unambiguous range',
'meta_group': 'instrument_parameters',
'long_name': 'Unambiguous range',
'data': array([467000., 467000., 467000., ..., 117000., 117000., 117000.],
dtype=float32)},
'nyquist_velocity': {'units': 'meters_per_second',
'comments': 'Unambiguous velocity',
'meta_group': 'instrument_parameters',
'long_name': 'Nyquist velocity',
'data': array([ 8.4 , 8.4 , 8.4 , ..., 33.56, 33.56, 33.56], dtype=float32)}},
'radar_calibration': None,
'ngates': 1832,
'nrays': 7920,
'nsweeps': 16,
'projection': {'proj': 'pyart_aeqd', '_include_lon_0_lat_0': True},
'rays_per_sweep': <pyart.lazydict.LazyLoadDict at 0x147e7ece8fb0>,
'gate_x': <pyart.lazydict.LazyLoadDict at 0x147e66a91cd0>,
'gate_y': <pyart.lazydict.LazyLoadDict at 0x147e66a91ee0>,
'gate_z': <pyart.lazydict.LazyLoadDict at 0x147e66a91f70>,
'gate_longitude': <pyart.lazydict.LazyLoadDict at 0x147e66a92000>,
'gate_latitude': <pyart.lazydict.LazyLoadDict at 0x147e66a92090>,
'gate_altitude': <pyart.lazydict.LazyLoadDict at 0x147e66a92120>}
We can get a list of all the individual keys in the dictionary:
radarDict.keys()
dict_keys(['time', 'range', 'fields', 'metadata', 'scan_type', 'latitude', 'longitude', 'altitude', 'altitude_agl', 'sweep_number', 'sweep_mode', 'fixed_angle', 'sweep_start_ray_index', 'sweep_end_ray_index', 'target_scan_rate', 'rays_are_indexed', 'ray_angle_res', 'azimuth', 'elevation', 'scan_rate', 'antenna_transition', 'rotation', 'tilt', 'roll', 'drift', 'heading', 'pitch', 'georefs_applied', 'instrument_parameters', 'radar_calibration', 'ngates', 'nrays', 'nsweeps', 'projection', 'rays_per_sweep', 'gate_x', 'gate_y', 'gate_z', 'gate_longitude', 'gate_latitude', 'gate_altitude'])
The first key is time
. Let’s look at it:
radarDict['time']
{'units': 'seconds since 2025-04-26T17:05:35Z',
'standard_name': 'time',
'long_name': 'time_in_seconds_since_volume_start',
'calendar': 'gregorian',
'comment': 'Coordinate variable for time. Time at the center of each ray, in fractional seconds since the global variable time_coverage_start',
'data': array([ 0.516, 0.556, 0.599, ..., 327.1 , 327.136, 327.174])}
It’s also a Python dictionary! It’s shorter than its parent’s … so we can see that there are six keys. Let’s look at the first one, units
.
radarDict['time']['units']
'seconds since 2025-04-26T17:05:35Z'
It’s a string … and a useful one … as it provides a base time that we can use to infer timestamps for the start of each of the individual sweeps that make up the full volume scan of this particular radar file.
Let’s look at time
’s data
key:
radar.time['data'] # equivalent to: radarDict['time']['data']
array([ 0.516, 0.556, 0.599, ..., 327.1 , 327.136, 327.174])
radar
dictionary can be accessed as an attribute in the radar
object itself. How many elements are in this array?
len(radar.time['data'])
7920
Other keys include longitude
and latitude
. Let’s have a look at them:
radar.longitude, radar.latitude
({'long_name': 'Longitude',
'standard_name': 'Longitude',
'units': 'degrees_east',
'data': array([-74.06408691])},
{'long_name': 'Latitude',
'standard_name': 'Latitude',
'units': 'degrees_north',
'data': array([42.58655548])})
These are also dictionaries! What do you think the data
keys represent in both of them?
Get the central longitude and latitude for the scan, which should correspond to the coordinates of the radar site. Older data may have these coordinates set to 0. If so, get the coordinates from a table, and update the radar
object.
def verify_radar_latlon (radar):
if radar.longitude['data'] == 0 or radar.latitude['data'] == 0:
print (f"No latitude/longitude for {site}; will get them from NEXRAD site table")
nexrad_sites = pd.read_csv('/spare11/atm350/data/nexrad_sites.csv')
site_row = nexrad_sites.query("siteID == @site")
radar.longitude['data'], radar.latitude['data'] = site_row['X'].values, site_row['Y'].values
return radar
radar = verify_radar_latlon(radar)
Let’s explore the sweep_number
, elevation
and fixed_angle
keys.
radarDict['sweep_number']
{'units': 'count',
'standard_name': 'sweep_number',
'long_name': 'Sweep number',
'data': array([ 0, 1, 2, 3, 4, 5, 6, 7, 8, 9, 10, 11, 12, 13, 14, 15],
dtype=int32)}
radarDict['elevation']
{'units': 'degrees',
'standard_name': 'beam_elevation_angle',
'long_name': 'elevation_angle_from_horizontal_plane',
'axis': 'radial_elevation_coordinate',
'comment': 'Elevation of antenna relative to the horizontal plane',
'data': array([ 0.64819336, 0.6069946 , 0.5657959 , ..., 13.974609 ,
13.974609 , 13.974609 ], dtype=float32)}
radarDict['elevation']['data']
array([ 0.64819336, 0.6069946 , 0.5657959 , ..., 13.974609 ,
13.974609 , 13.974609 ], dtype=float32)
What is the length of the data array associated with elevation?
len(radarDict['elevation']['data'])
7920
radarDict['fixed_angle']['data']
array([ 0.48339844, 0.48339844, 0.87890625, 0.87890625, 1.3183594 ,
1.3183594 , 1.8017578 , 2.4169922 , 3.1201172 , 3.9990234 ,
5.0976562 , 6.4160156 , 7.998047 , 10.019531 , 11.99707 ,
14.018555 ], dtype=float32)
Notice the first several fixed-elevation angles may be duplicated!
Let’s look at the number of rays in each sweep.
ssri = radarDict['sweep_start_ray_index']
ssri
{'long_name': 'Index of first ray in sweep, 0-based',
'units': 'count',
'data': array([ 0, 720, 1440, 2160, 2880, 3600, 4320, 4680, 5040, 5400, 5760,
6120, 6480, 6840, 7200, 7560], dtype=int32)}
seri = radarDict['sweep_end_ray_index']
seri
{'long_name': 'Index of last ray in sweep, 0-based',
'units': 'count',
'data': array([ 719, 1439, 2159, 2879, 3599, 4319, 4679, 5039, 5399, 5759, 6119,
6479, 6839, 7199, 7559, 7919], dtype=int32)}
seri['data'] - ssri['data'] + 1
array([720, 720, 720, 720, 720, 720, 360, 360, 360, 360, 360, 360, 360,
360, 360, 360], dtype=int32)
cLon, cLat = radar.longitude['data'], radar.latitude['data']
Specify latitude and longitude bounds for the resulting maps, the resolution of the cartographic shapefiles, and the desired sweep level.
lonW = cLon - 2
lonE = cLon + 2
latS = cLat - 2
latN = cLat + 2
domain = lonW, lonE, latS, latN
res = '10m'
sweep = 0
Define a function that will determine at which ray a particular sweep begins; also define some strings for the figure title.
def nexRadSweepTimeElev (radar, sweep):
sweepRayIndex = radar.sweep_start_ray_index['data'][sweep]
baseTimeStr = radar.time['units'].split()[-1]
baseTime = dt.strptime(baseTimeStr, "%Y-%m-%dT%H:%M:%SZ")
timeSweep = baseTime + timedelta(seconds=radar.time['data'][sweepRayIndex])
timeSweepStr = dt.strftime(timeSweep, format="%Y-%m-%d %H:%M:%S UTC")
elevSweep = radar.fixed_angle['data'][sweep]
elevSweepStr = f'{elevSweep:.1f}°'
return timeSweepStr, elevSweepStr
radar.sweep_start_ray_index
{'long_name': 'Index of first ray in sweep, 0-based',
'units': 'count',
'data': array([ 0, 720, 1440, 2160, 2880, 3600, 4320, 4680, 5040, 5400, 5760,
6120, 6480, 6840, 7200, 7560], dtype=int32)}
radar.sweep_number
{'units': 'count',
'standard_name': 'sweep_number',
'long_name': 'Sweep number',
'data': array([ 0, 1, 2, 3, 4, 5, 6, 7, 8, 9, 10, 11, 12, 13, 14, 15],
dtype=int32)}
field = 'reflectivity'
shortName = 'REFL'
Create a single figure of reflectivity, zoomed into the area of interest.#
# Creating color tables for reflectivity (every 5 dBZ starting with 5 dBZ):
ref_norm, ref_cmap = ctables.registry.get_with_steps('NWSReflectivity', 5, 5)
ref_cmap
We’ll add range rings to the display.#
# Call the function that creates the title string, among other things.
timeSweepStr, elevSweepStr = nexRadSweepTimeElev (radar, sweep)
titleStr = f'{site} {shortName} {elevSweepStr} {timeSweepStr}'
# Create our figure
fig = plt.figure(figsize=[15, 6])
# Set up a single axes and plot reflectivity
ax = plt.subplot(111, projection=ccrs.PlateCarree())
ax.set_extent ([lonW, lonE, latS, latN])
display = pyart.graph.RadarMapDisplay(radar)
ref_map = display.plot_ppi_map(field,sweep=sweep, vmin=20, vmax=80, ax=ax, raster=False, title=titleStr,
colorbar_label='Equivalent Relectivity ($Z_{e}$) (dBZ)', norm=ref_norm, cmap=ref_cmap, resolution=res)
range_rings = display.plot_range_rings([10,50,100,200],ax=ax, col='brown',ls='dashed',lw=0.5)
# Add counties
ax.add_feature(USCOUNTIES, linewidth=0.5);
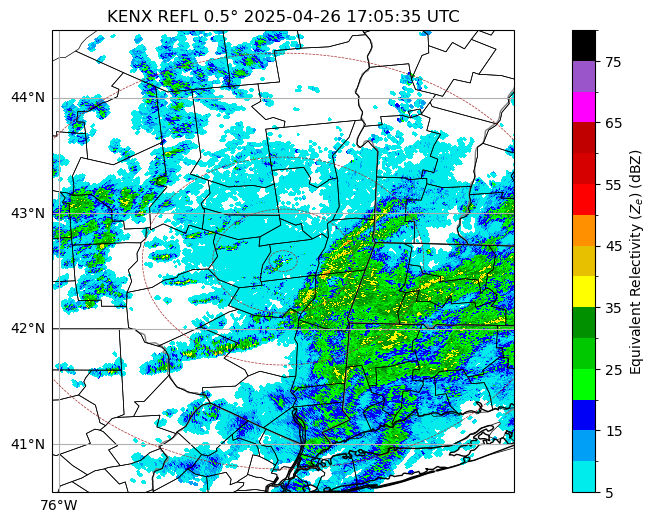
Next, let’s create a function to create a plot, so we can loop over all the radar files of the specified hour.
def plot_radar_refl (idx, site, radar):
proj = ccrs.PlateCarree()
# New axes with the specified projection
ax = fig.add_subplot(111, projection=proj)
ax.set_extent(domain)
ax.add_feature(USCOUNTIES.with_scale('5m'),edgecolor='grey', linewidth=1, zorder = 3 )
display = pyart.graph.RadarMapDisplay(radar)
ref_map = display.plot_ppi_map(field,sweep=sweep, cmap=ref_cmap, norm=ref_norm, ax=ax, colorbar_flag = False,
title_flag = False, colorbar_label='Equivalent Relectivity ($Z_{e}$) (dBZ)', resolution=res)
return ax
Loop over the files. Save each image.
backend_ = matplotlib.get_backend()
backend_
'module://matplotlib_inline.backend_inline'
Set the Jupyter matplotlib backend to one that will not show the graphics inline (this will save time in notebook execution)
Set an increment for how many individual frames we should skip. In general, this should be set to the same value as the number of hours, in order to keep the animation size as well as the time to create the animation manageable.#
incr = nHours
matplotlib.use("Agg") # Prevent showing stuff
meshes = []
fig = plt.figure(figsize=(10,10))
for n, name in enumerate(fileList[::nHours]):
print (n, name, site)
radar = pyart.io.read_nexrad_archive(f's3://{name}')
radar = verify_radar_latlon(radar)
ax1 = plot_radar_refl(n, site, radar)
timeSweepStr, elevSweepStr = nexRadSweepTimeElev (radar, sweep)
titleStr = f'{site} {shortName} {elevSweepStr} {timeSweepStr}'
print (titleStr)
title = ax1.text(0.5,0.92,titleStr,horizontalalignment='center',
verticalalignment='center', transform=fig.transFigure, fontsize=15)
meshes.append((ax1,title))
0 noaa-nexrad-level2/2025/04/26/KENX/KENX20250426_170535_V06 KENX
KENX REFL 0.5° 2025-04-26 17:05:35 UTC
1 noaa-nexrad-level2/2025/04/26/KENX/KENX20250426_172728_V06 KENX
KENX REFL 0.5° 2025-04-26 17:27:28 UTC
2 noaa-nexrad-level2/2025/04/26/KENX/KENX20250426_174723_V06 KENX
KENX REFL 0.5° 2025-04-26 17:47:23 UTC
3 noaa-nexrad-level2/2025/04/26/KENX/KENX20250426_180607_V06 KENX
KENX REFL 0.5° 2025-04-26 18:06:07 UTC
4 noaa-nexrad-level2/2025/04/26/KENX/KENX20250426_182505_V06 KENX
KENX REFL 0.5° 2025-04-26 18:25:05 UTC
5 noaa-nexrad-level2/2025/04/26/KENX/KENX20250426_184202_V06 KENX
KENX REFL 0.5° 2025-04-26 18:42:02 UTC
6 noaa-nexrad-level2/2025/04/26/KENX/KENX20250426_190013_V06 KENX
KENX REFL 0.5° 2025-04-26 19:00:13 UTC
7 noaa-nexrad-level2/2025/04/26/KENX/KENX20250426_191906_V06 KENX
KENX REFL 0.5° 2025-04-26 19:19:06 UTC
8 noaa-nexrad-level2/2025/04/26/KENX/KENX20250426_193746_V06 KENX
KENX REFL 0.5° 2025-04-26 19:37:46 UTC
9 noaa-nexrad-level2/2025/04/26/KENX/KENX20250426_195612_V06 KENX
KENX REFL 0.5° 2025-04-26 19:56:12 UTC
10 noaa-nexrad-level2/2025/04/26/KENX/KENX20250426_201404_V06 KENX
KENX REFL 0.5° 2025-04-26 20:14:04 UTC
11 noaa-nexrad-level2/2025/04/26/KENX/KENX20250426_203119_V06 KENX
KENX REFL 0.5° 2025-04-26 20:31:19 UTC
12 noaa-nexrad-level2/2025/04/26/KENX/KENX20250426_204820_V06 KENX
KENX REFL 0.5° 2025-04-26 20:48:20 UTC
Set the Jupyter matplotlib backend to the default value, so we see output once again.
matplotlib.use(backend_)
anim = ArtistAnimation(fig, meshes)
Display the animation (this may take some time, depending on the number of frames)
anim
Save the animation to the current directory (this may also take some time)
anim.save(f'{site}_{start_time_str}_refl.mp4')
Things to try#
Create an animation for a time and site of interest. Download the mp4 file and try loading it in Powerpoint.