Surface map of METAR data
Contents
Surface map of METAR data¶
Objective¶
In this notebook, we will make a surface map based on current observations from METAR sites across North America.
from datetime import datetime,timedelta
import numpy as np
import pandas as pd
import matplotlib.pyplot as plt
import cartopy.crs as ccrs
import cartopy.feature as cfeature
from metpy.calc import wind_components, reduce_point_density
from metpy.units import units
from metpy.plots import StationPlot
from metpy.plots.wx_symbols import current_weather, sky_cover, wx_code_map
# Load in a collection of functions that process GEMPAK weather conditions and cloud cover data.
%run /kt11/ktyle/python/metargem.py
Determine the date and hour to gather observations ¶
# Use the current time, or set your own for a past time.
# Set current to False if you want to specify a past time.
nowTime = datetime.utcnow()
#current = True
current = False
if (current):
validTime = datetime.utcnow()
year = validTime.year
month = validTime.month
day = validTime.day
hour = validTime.hour
else:
year = 2024
month = 3
day = 23
hour = 23
validTime = datetime(year, month, day, hour)
deltaTime = nowTime - validTime
deltaDays = deltaTime.days
timeStr = validTime.strftime("%Y-%m-%d %H UTC")
timeStr2 = validTime.strftime("%Y%m%d%H")
YYMMDDHH = validTime.strftime("%y%m%d%H")
print(timeStr)
print(validTime)
print(deltaTime)
print(deltaDays)
2024-03-23 23 UTC
2024-03-23 23:00:00
37 days, 1:59:10.895662
37
The METAR data are in hourly CSV files which can be opened by Pandas.¶
Top
metarFile = f'/ktyle_rit/scripts/sflist2/complete/{YYMMDDHH}.csv'
df = pd.read_csv(metarFile, sep="\s+")
df
STN | YYMMDD/HHMM | SLAT | SLON | SELV | PMSL | ALTI | TMPC | DWPC | SKNT | ... | P03C | CTYL | CTYM | CTYH | P06I | T6XC | T6NC | CEIL | P01I | SNEW | |
---|---|---|---|---|---|---|---|---|---|---|---|---|---|---|---|---|---|---|---|---|---|
0 | DYS | 240323/2300 | 32.43 | -99.85 | 545.0 | 1010.2 | 29.89 | 20.8 | 10.9 | 18.0 | ... | -9999.0 | -9999.0 | -9999.0 | -9999.0 | -9999.0 | -9999.0 | -9999.0 | -9999.0 | -9999.00 | -9999.0 |
1 | NUW | 240323/2300 | 48.35 | -122.65 | 14.0 | 1002.0 | 29.58 | 8.9 | 7.2 | 5.0 | ... | -9999.0 | -9999.0 | -9999.0 | -9999.0 | -9999.0 | -9999.0 | -9999.0 | 35.0 | 0.05 | -9999.0 |
2 | NYL | 240323/2300 | 32.65 | -114.62 | 65.0 | 1004.6 | 29.67 | 26.7 | 0.6 | 7.0 | ... | -9999.0 | -9999.0 | -9999.0 | -9999.0 | -9999.0 | -9999.0 | -9999.0 | -9999.0 | -9999.00 | -9999.0 |
3 | PALU | 240323/2300 | 68.88 | -166.13 | 3.0 | 1011.6 | 29.87 | -12.9 | -14.8 | 1.0 | ... | -9999.0 | -9999.0 | -9999.0 | -9999.0 | -9999.0 | -9999.0 | -9999.0 | 14.0 | 0.00 | -9999.0 |
4 | PATC | 240323/2300 | 65.57 | -167.92 | 83.0 | 1007.6 | 29.72 | -13.8 | -14.9 | 26.0 | ... | -9999.0 | -9999.0 | -9999.0 | -9999.0 | -9999.0 | -9999.0 | -9999.0 | 2.0 | 0.00 | -9999.0 |
... | ... | ... | ... | ... | ... | ... | ... | ... | ... | ... | ... | ... | ... | ... | ... | ... | ... | ... | ... | ... | ... |
4297 | 60R | 240323/2300 | -9999.00 | -9999.00 | -9999.0 | 1006.5 | 29.96 | 23.9 | 14.2 | 3.0 | ... | -9999.0 | -9999.0 | -9999.0 | -9999.0 | -9999.0 | -9999.0 | -9999.0 | -9999.0 | -9999.00 | -9999.0 |
4298 | 74V | 240323/2300 | -9999.00 | -9999.00 | -9999.0 | -9999.0 | 29.58 | 13.0 | -2.0 | 12.0 | ... | -9999.0 | -9999.0 | -9999.0 | -9999.0 | -9999.0 | -9999.0 | -9999.0 | 85.0 | -9999.00 | -9999.0 |
4299 | MOR | 240323/2300 | -9999.00 | -9999.00 | -9999.0 | -9999.0 | 30.03 | 10.0 | 1.0 | 5.0 | ... | -9999.0 | -9999.0 | -9999.0 | -9999.0 | -9999.0 | -9999.0 | -9999.0 | -9999.0 | -9999.00 | -9999.0 |
4300 | E57 | 240323/2300 | -9999.00 | -9999.00 | -9999.0 | -9999.0 | 29.85 | 21.0 | 4.0 | 18.0 | ... | -9999.0 | -9999.0 | -9999.0 | -9999.0 | -9999.0 | -9999.0 | -9999.0 | -9999.0 | -9999.00 | -9999.0 |
4301 | 1HW | 240323/2300 | -9999.00 | -9999.00 | -9999.0 | 1005.8 | 29.64 | 6.4 | -1.3 | 17.0 | ... | -9999.0 | -9999.0 | -9999.0 | -9999.0 | -9999.0 | -9999.0 | -9999.0 | -9999.0 | -9999.00 | -9999.0 |
4302 rows × 35 columns
Set the domain to gather data from and for defining the plot region.¶
latN = 55
latS = 20
lonW = -125
lonE = -60
cLat = (latN + latS)/2
cLon = (lonW + lonE )/2
Perform the spatial subset. Extend the data bounds slightly.
extend = 0.01
df2 = df.query('SLAT >= (@latS - @extend) & SLAT <= (@latN + @extend) & SLON >= (@lonW - @extend) & SLON <= (@lonE + @extend)')
df2
STN | YYMMDD/HHMM | SLAT | SLON | SELV | PMSL | ALTI | TMPC | DWPC | SKNT | ... | P03C | CTYL | CTYM | CTYH | P06I | T6XC | T6NC | CEIL | P01I | SNEW | |
---|---|---|---|---|---|---|---|---|---|---|---|---|---|---|---|---|---|---|---|---|---|
0 | DYS | 240323/2300 | 32.43 | -99.85 | 545.0 | 1010.2 | 29.89 | 20.8 | 10.9 | 18.0 | ... | -9999.0 | -9999.0 | -9999.0 | -9999.0 | -9999.0 | -9999.0 | -9999.0 | -9999.0 | -9999.00 | -9999.0 |
1 | NUW | 240323/2300 | 48.35 | -122.65 | 14.0 | 1002.0 | 29.58 | 8.9 | 7.2 | 5.0 | ... | -9999.0 | -9999.0 | -9999.0 | -9999.0 | -9999.0 | -9999.0 | -9999.0 | 35.0 | 0.05 | -9999.0 |
2 | NYL | 240323/2300 | 32.65 | -114.62 | 65.0 | 1004.6 | 29.67 | 26.7 | 0.6 | 7.0 | ... | -9999.0 | -9999.0 | -9999.0 | -9999.0 | -9999.0 | -9999.0 | -9999.0 | -9999.0 | -9999.00 | -9999.0 |
9 | CACQ | 240323/2300 | 47.00 | -65.45 | 34.0 | 1022.5 | -9999.00 | -6.0 | -7.0 | 3.0 | ... | -9999.0 | -9999.0 | -9999.0 | -9999.0 | -9999.0 | -9999.0 | -9999.0 | -9999.0 | -9999.00 | -9999.0 |
10 | CAFC | 240323/2300 | 45.92 | -66.60 | 35.0 | 1019.2 | -9999.00 | -5.0 | -6.0 | 6.0 | ... | -9999.0 | -9999.0 | -9999.0 | -9999.0 | -9999.0 | -9999.0 | -9999.0 | -9999.0 | -9999.00 | -9999.0 |
... | ... | ... | ... | ... | ... | ... | ... | ... | ... | ... | ... | ... | ... | ... | ... | ... | ... | ... | ... | ... | ... |
4190 | 5T9 | 240323/2300 | 28.86 | -100.51 | 270.0 | -9999.0 | 29.88 | -9999.0 | -9999.0 | 13.0 | ... | -9999.0 | -9999.0 | -9999.0 | -9999.0 | -9999.0 | -9999.0 | -9999.0 | -9999.0 | -9999.00 | -9999.0 |
4191 | CPU | 240323/2300 | 38.15 | -120.65 | 404.0 | -9999.0 | 29.85 | 8.0 | 7.1 | 5.0 | ... | -9999.0 | -9999.0 | -9999.0 | -9999.0 | -9999.0 | -9999.0 | -9999.0 | -9999.0 | -9999.00 | -9999.0 |
4192 | 3LF | 240323/2300 | 39.16 | -89.67 | 210.0 | -9999.0 | 30.21 | 7.3 | -5.4 | 8.0 | ... | -9999.0 | -9999.0 | -9999.0 | -9999.0 | -9999.0 | -9999.0 | -9999.0 | -9999.0 | -9999.00 | -9999.0 |
4193 | NJW | 240323/2300 | 32.80 | -88.83 | 165.0 | 1008.8 | 30.04 | 17.8 | 3.9 | 11.0 | ... | -9999.0 | -9999.0 | -9999.0 | -9999.0 | -9999.0 | -9999.0 | -9999.0 | -9999.0 | -9999.00 | -9999.0 |
4194 | 2DP | 240323/2300 | 35.68 | -75.90 | 3.0 | -9999.0 | 29.69 | 14.2 | 12.9 | 6.0 | ... | -9999.0 | -9999.0 | -9999.0 | -9999.0 | -9999.0 | -9999.0 | -9999.0 | -9999.0 | -9999.00 | -9999.0 |
2686 rows × 35 columns
Select the weather variables of interest. Also include the site ID, lat, lon, elevation, and time columns.
columnSubset = ['STN', 'YYMMDD/HHMM', 'SLAT', 'SLON', 'SELV', 'TMPC', 'DWPC', 'PMSL',
'SKNT', 'DRCT','ALTI','WNUM','VSBY','CHC1', 'CHC2', 'CHC3','CTYH', 'CTYM', 'CTYL']
df3 = df2[columnSubset]
df3
STN | YYMMDD/HHMM | SLAT | SLON | SELV | TMPC | DWPC | PMSL | SKNT | DRCT | ALTI | WNUM | VSBY | CHC1 | CHC2 | CHC3 | CTYH | CTYM | CTYL | |
---|---|---|---|---|---|---|---|---|---|---|---|---|---|---|---|---|---|---|---|
0 | DYS | 240323/2300 | 32.43 | -99.85 | 545.0 | 20.8 | 10.9 | 1010.2 | 18.0 | 140.0 | 29.89 | -9999.0 | 10.0 | 1.0 | -9999.0 | -9999.0 | -9999.0 | -9999.0 | -9999.0 |
1 | NUW | 240323/2300 | 48.35 | -122.65 | 14.0 | 8.9 | 7.2 | 1002.0 | 5.0 | 320.0 | 29.58 | 13.0 | 8.0 | 206.0 | 353.0 | 504.0 | -9999.0 | -9999.0 | -9999.0 |
2 | NYL | 240323/2300 | 32.65 | -114.62 | 65.0 | 26.7 | 0.6 | 1004.6 | 7.0 | 230.0 | 29.67 | -9999.0 | 10.0 | 1106.0 | -9999.0 | -9999.0 | -9999.0 | -9999.0 | -9999.0 |
9 | CACQ | 240323/2300 | 47.00 | -65.45 | 34.0 | -6.0 | -7.0 | 1022.5 | 3.0 | 50.0 | -9999.00 | -9999.0 | -9999.0 | -9999.0 | -9999.0 | -9999.0 | -9999.0 | -9999.0 | -9999.0 |
10 | CAFC | 240323/2300 | 45.92 | -66.60 | 35.0 | -5.0 | -6.0 | 1019.2 | 6.0 | 30.0 | -9999.00 | -9999.0 | -9999.0 | -9999.0 | -9999.0 | -9999.0 | -9999.0 | -9999.0 | -9999.0 |
... | ... | ... | ... | ... | ... | ... | ... | ... | ... | ... | ... | ... | ... | ... | ... | ... | ... | ... | ... |
4190 | 5T9 | 240323/2300 | 28.86 | -100.51 | 270.0 | -9999.0 | -9999.0 | -9999.0 | 13.0 | 120.0 | 29.88 | -9999.0 | 10.0 | 1.0 | -9999.0 | -9999.0 | -9999.0 | -9999.0 | -9999.0 |
4191 | CPU | 240323/2300 | 38.15 | -120.65 | 404.0 | 8.0 | 7.1 | -9999.0 | 5.0 | 140.0 | 29.85 | -9999.0 | 10.0 | 162.0 | 252.0 | 452.0 | -9999.0 | -9999.0 | -9999.0 |
4192 | 3LF | 240323/2300 | 39.16 | -89.67 | 210.0 | 7.3 | -5.4 | -9999.0 | 8.0 | 40.0 | 30.21 | -9999.0 | 10.0 | 1.0 | -9999.0 | -9999.0 | -9999.0 | -9999.0 | -9999.0 |
4193 | NJW | 240323/2300 | 32.80 | -88.83 | 165.0 | 17.8 | 3.9 | 1008.8 | 11.0 | 360.0 | 30.04 | -9999.0 | 10.0 | 1.0 | -9999.0 | -9999.0 | -9999.0 | -9999.0 | -9999.0 |
4194 | 2DP | 240323/2300 | 35.68 | -75.90 | 3.0 | 14.2 | 12.9 | -9999.0 | 6.0 | 30.0 | 29.69 | -9999.0 | 10.0 | 1.0 | -9999.0 | -9999.0 | -9999.0 | -9999.0 | -9999.0 |
2686 rows × 19 columns
In these datasets, -9999.0 signfies missing values. Replace all instances of -9999.0 with NumPy’s NaN
(not a number) value.
df4 = df3.replace(-9999.0, np.nan)
df4
STN | YYMMDD/HHMM | SLAT | SLON | SELV | TMPC | DWPC | PMSL | SKNT | DRCT | ALTI | WNUM | VSBY | CHC1 | CHC2 | CHC3 | CTYH | CTYM | CTYL | |
---|---|---|---|---|---|---|---|---|---|---|---|---|---|---|---|---|---|---|---|
0 | DYS | 240323/2300 | 32.43 | -99.85 | 545.0 | 20.8 | 10.9 | 1010.2 | 18.0 | 140.0 | 29.89 | NaN | 10.0 | 1.0 | NaN | NaN | NaN | NaN | NaN |
1 | NUW | 240323/2300 | 48.35 | -122.65 | 14.0 | 8.9 | 7.2 | 1002.0 | 5.0 | 320.0 | 29.58 | 13.0 | 8.0 | 206.0 | 353.0 | 504.0 | NaN | NaN | NaN |
2 | NYL | 240323/2300 | 32.65 | -114.62 | 65.0 | 26.7 | 0.6 | 1004.6 | 7.0 | 230.0 | 29.67 | NaN | 10.0 | 1106.0 | NaN | NaN | NaN | NaN | NaN |
9 | CACQ | 240323/2300 | 47.00 | -65.45 | 34.0 | -6.0 | -7.0 | 1022.5 | 3.0 | 50.0 | NaN | NaN | NaN | NaN | NaN | NaN | NaN | NaN | NaN |
10 | CAFC | 240323/2300 | 45.92 | -66.60 | 35.0 | -5.0 | -6.0 | 1019.2 | 6.0 | 30.0 | NaN | NaN | NaN | NaN | NaN | NaN | NaN | NaN | NaN |
... | ... | ... | ... | ... | ... | ... | ... | ... | ... | ... | ... | ... | ... | ... | ... | ... | ... | ... | ... |
4190 | 5T9 | 240323/2300 | 28.86 | -100.51 | 270.0 | NaN | NaN | NaN | 13.0 | 120.0 | 29.88 | NaN | 10.0 | 1.0 | NaN | NaN | NaN | NaN | NaN |
4191 | CPU | 240323/2300 | 38.15 | -120.65 | 404.0 | 8.0 | 7.1 | NaN | 5.0 | 140.0 | 29.85 | NaN | 10.0 | 162.0 | 252.0 | 452.0 | NaN | NaN | NaN |
4192 | 3LF | 240323/2300 | 39.16 | -89.67 | 210.0 | 7.3 | -5.4 | NaN | 8.0 | 40.0 | 30.21 | NaN | 10.0 | 1.0 | NaN | NaN | NaN | NaN | NaN |
4193 | NJW | 240323/2300 | 32.80 | -88.83 | 165.0 | 17.8 | 3.9 | 1008.8 | 11.0 | 360.0 | 30.04 | NaN | 10.0 | 1.0 | NaN | NaN | NaN | NaN | NaN |
4194 | 2DP | 240323/2300 | 35.68 | -75.90 | 3.0 | 14.2 | 12.9 | NaN | 6.0 | 30.0 | 29.69 | NaN | 10.0 | 1.0 | NaN | NaN | NaN | NaN | NaN |
2686 rows × 19 columns
data = df4
In our current data archive, multiple weather types are often not represented properly. To avoid a type conversion error, set stations whose weather type fall into this category to missing.
This will eventually be fixed!
data.loc[data['WNUM'] =='********', ['WNUM']] = '-9999.00'
data['WNUM'] = data['WNUM'].astype('float16')
/tmp/ipykernel_1786001/3580734062.py:1: FutureWarning: Setting an item of incompatible dtype is deprecated and will raise in a future error of pandas. Value '-9999.00' has dtype incompatible with float64, please explicitly cast to a compatible dtype first.
data.loc[data['WNUM'] =='********', ['WNUM']] = '-9999.00'
Read in several of the columns as Pandas Series
; extract the value arrays from the Series
objects; assign / convert units as necessary; convert GEMPAK cloud cover and present wx symbols to MetPy’s representation¶
lats = data['SLAT'].values
lons = data['SLON'].values
tair = (data['TMPC'].values * units ('degC')).to('degF')
dewp = (data['DWPC'].values * units ('degC')).to('degF')
altm = (data['ALTI'].values * units('inHg')).to('mbar')
slp = data['PMSL'].values * units('hPa')
# Convert wind to components
u, v = wind_components(data['SKNT'].values * units.knots, data['DRCT'].values * units.degree)
# replace missing wx codes or those >= 100 with 0, and convert to MetPy's present weather code
wnum = (np.nan_to_num(data['WNUM'].values,True).astype(int))
convert_wnum (wnum)
# Need to handle missing (NaN) and convert to proper code
chc1 = (np.nan_to_num(data['CHC1'].values,True).astype(int))
chc2 = (np.nan_to_num(data['CHC2'].values,True).astype(int))
chc3 = (np.nan_to_num(data['CHC3'].values,True).astype(int))
cloud_cover = calc_clouds(chc1, chc2, chc3)
# For some reason station id's come back as bytes instead of strings. This line converts the array of station id's into an array of strings.
#stid = np.array([s.decode() for s in data['station']])
stid = data['STN']
The next step deals with the removal of overlapping stations, using reduce_point_density
. This returns a mask we can apply to data to filter the points.
Select a Cartopy map projection, which we will use both to reduce plotted station density and to plot the data on a map.
# Uncomment whichever projection you want (or add your own!)
# proj = ccrs.Stereographic(central_longitude=cLon, central_latitude=cLat)
proj = ccrs.LambertConformal(central_longitude=cLon, central_latitude=cLat)
# Project points so that we're filtering based on the way the stations are laid out on the map
xy = proj.transform_points(ccrs.PlateCarree(), lons, lats)
# Reduce point density so that there's only one point within a circle whose distance is specified in meters.
# This value will need to change depending on how large of an area you are plotting.
density = 150000
mask = reduce_point_density(xy, density)
transform_points
, we pass in the arrays of the stations' longitudes and latitudes. Since their units are in degrees, we need to also specify their corresponding map projection ... which is PlateCarree for degrees of longitude and latitude.Visualize Surface Observations ¶
Top
Simple station plotting using plot methods¶
One way to create station plots with MetPy is to create an instance of StationPlot
and call various plot methods, like plot_parameter
, to plot arrays of data at locations relative to the center point.
In addition to plotting values, StationPlot
has support for plotting text strings, symbols, and plotting values using custom formatting.
Plotting symbols involves mapping integer values to various custom font glyphs in our custom weather symbols font. MetPy provides mappings for converting WMO codes to their appropriate symbol. The sky_cover
and current_weather
functions below are two such mappings.
Now we just plot with arr[mask]
for every arr
of data we use in plotting.
# Set up a plot with map features
# First set dpi ("dots per inch") - higher values will give us a less pixelated final figure.
dpi = 125
fig = plt.figure(figsize=(24,18), dpi=dpi)
ax = fig.add_subplot(1, 1, 1, projection=proj) # We use the projection defined above
ax.set_facecolor(cfeature.COLORS['water'])
land_mask = cfeature.NaturalEarthFeature('physical', 'land', '50m',
edgecolor='face',
facecolor=cfeature.COLORS['land'])
lake_mask = cfeature.NaturalEarthFeature('physical', 'lakes', '50m',
edgecolor='face',
facecolor=cfeature.COLORS['water'])
state_borders = cfeature.NaturalEarthFeature(category='cultural', name='admin_1_states_provinces_lakes',
scale='50m', facecolor='none')
ax.add_feature(land_mask)
ax.add_feature(lake_mask)
ax.add_feature(state_borders, linestyle='solid', edgecolor='black')
# Slightly reduce the extent of the map as compared to the subsetted data region; this helps eliminate data from being plotted beyond the frame of the map
ax.set_extent ((lonW+2,lonE-4,latS+1,latN-2), crs=ccrs.PlateCarree())
ax.set_extent ((lonW+0.2,lonE-0.2,latS+.1,latN-.1), crs=ccrs.PlateCarree())
ax.set_extent ((lonW,lonE,latS,latN), crs=ccrs.PlateCarree())
#If we wanted to add grid lines to our plot:
#ax.gridlines()
# Create a station plot pointing to an Axes to draw on as well as the location of points
stationplot = StationPlot(ax, lons[mask], lats[mask], transform=ccrs.PlateCarree(),
fontsize=8)
stationplot.plot_parameter('NW', tair[mask], color='red', fontsize=10)
stationplot.plot_parameter('SW', dewp[mask], color='darkgreen', fontsize=10)
# Below, we are using a custom formatter to control how the sea-level pressure
# values are plotted. This uses the standard trailing 3-digits of the pressure value
# in tenths of millibars.
stationplot.plot_parameter('NE', slp[mask], color='purple', formatter=lambda v: format(10 * v, '.0f')[-3:])
stationplot.plot_symbol('C', cloud_cover[mask], sky_cover)
stationplot.plot_symbol('W', wnum[mask], current_weather,color='blue',fontsize=12)
stationplot.plot_text((2, 0),stid[mask], color='gray')
#zorder - Higher value zorder will plot the variable on top of lower value zorder. This is necessary for wind barbs to appear. Default is 1.
stationplot.plot_barb(u[mask], v[mask],zorder=2)
plotTitle = (f"Sfc Map valid at: {timeStr}")
ax.set_title (plotTitle);
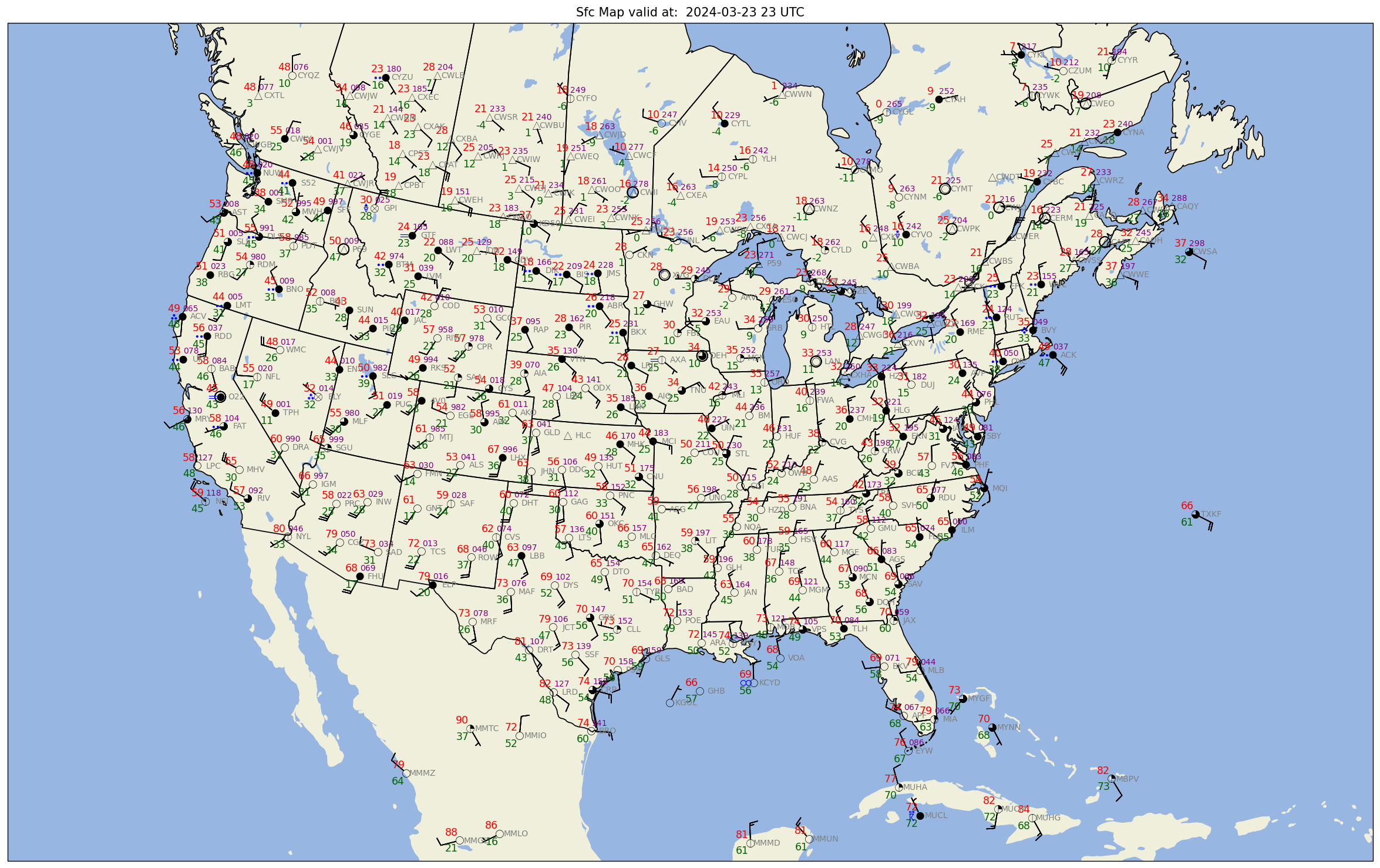
# In order to see the entire figure, type the name of the figure object below.
fig
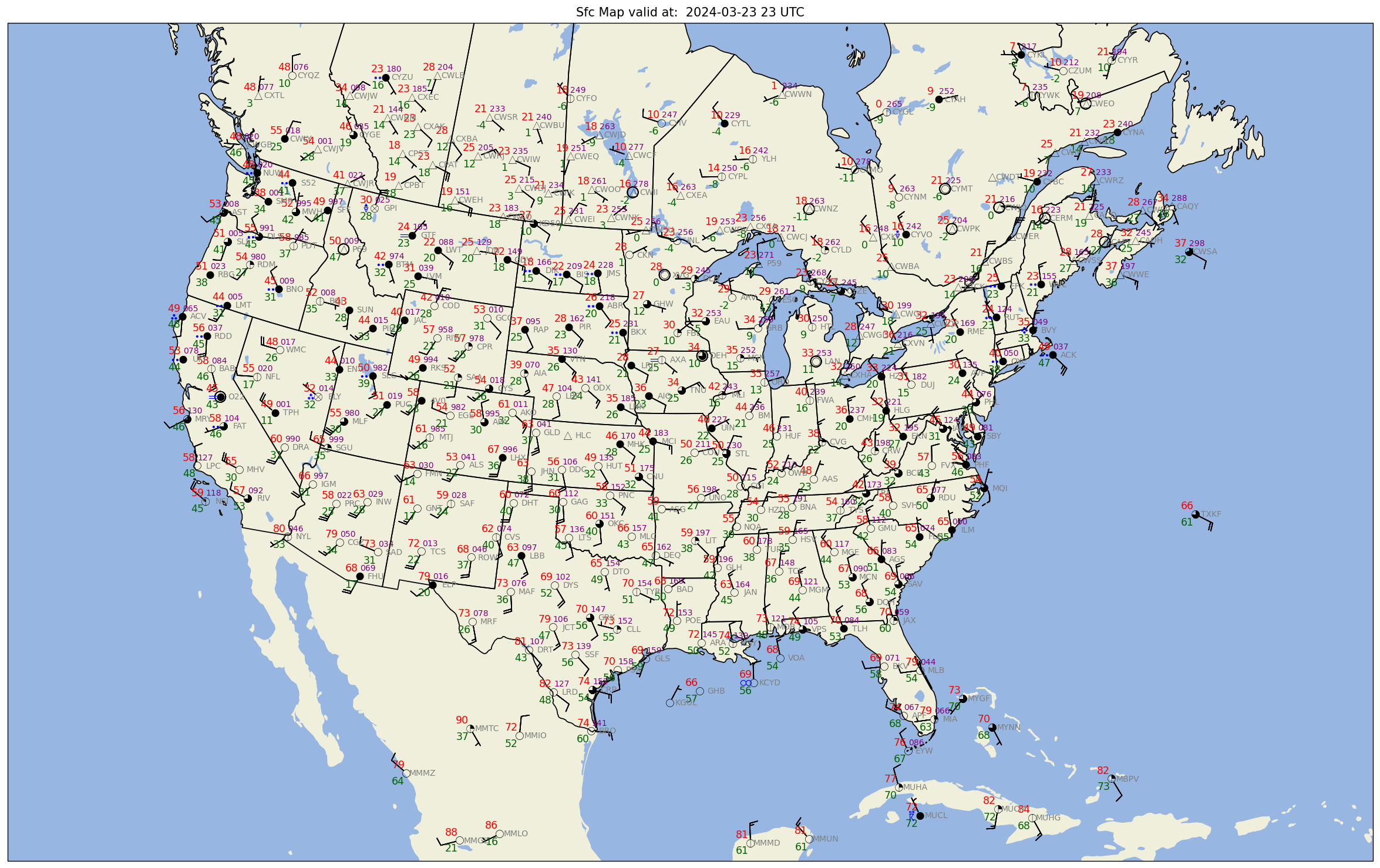
figName = (f'{timeStr2}_sfmap.png')
fig.savefig(figName)