MetPy Intro: NYS Mesonet Map
Contents
MetPy Intro: NYS Mesonet Map¶
Overview¶
In this notebook, we’ll use Cartopy, Matplotlib, and Pandas (with a bunch of help from MetPy) to read in, manipulate, and visualize current data from the New York State Mesonet.
Prerequisites¶
Concepts |
Importance |
Notes |
---|---|---|
Matplotlib |
Necessary |
|
Cartopy |
Necessary |
|
Pandas |
Necessary |
|
MetPy |
Necessary |
Intro |
Time to learn: 30 minutes
Imports¶
import matplotlib.pyplot as plt
import numpy as np
import pandas as pd
from datetime import datetime
from cartopy import crs as ccrs
from cartopy import feature as cfeature
from metpy.calc import wind_components, dewpoint_from_relative_humidity
from metpy.units import units
from metpy.plots import StationPlot, USCOUNTIES
Create a Pandas DataFrame
object pointing to the most recent set of NYSM obs.
nysm_data = pd.read_csv('https://www.atmos.albany.edu/products/nysm/nysm_latest.csv')
nysm_data.columns
Index(['station', 'time', 'temp_2m [degC]', 'temp_9m [degC]',
'relative_humidity [percent]', 'precip_incremental [mm]',
'precip_local [mm]', 'precip_max_intensity [mm/min]',
'avg_wind_speed_prop [m/s]', 'max_wind_speed_prop [m/s]',
'wind_speed_stddev_prop [m/s]', 'wind_direction_prop [degrees]',
'wind_direction_stddev_prop [degrees]', 'avg_wind_speed_sonic [m/s]',
'max_wind_speed_sonic [m/s]', 'wind_speed_stddev_sonic [m/s]',
'wind_direction_sonic [degrees]',
'wind_direction_stddev_sonic [degrees]', 'solar_insolation [W/m^2]',
'station_pressure [mbar]', 'snow_depth [cm]', 'frozen_soil_05cm [bit]',
'frozen_soil_25cm [bit]', 'frozen_soil_50cm [bit]',
'soil_temp_05cm [degC]', 'soil_temp_25cm [degC]',
'soil_temp_50cm [degC]', 'soil_moisture_05cm [m^3/m^3]',
'soil_moisture_25cm [m^3/m^3]', 'soil_moisture_50cm [m^3/m^3]', 'lat',
'lon', 'elevation', 'name'],
dtype='object')
Create several Series
objects for some of the columns.
stid = nysm_data['station']
lats = nysm_data['lat']
lons = nysm_data['lon']
Our goal is to make a map of NYSM observations, which includes the wind velocity. The convention is to plot wind velocity using wind barbs. The MetPy library allows us to not only make such a map, but perform a variety of meteorologically-relevant calculations and diagnostics. Here, we will use such a calculation, which will determine the two scalar components of wind velocity (u and v), from wind speed and direction. We will use MetPy’s wind_components method.
This method requires us to do the following:
Create Pandas
Series
objects for the variables of interestExtract the underlying Numpy array via the
Series
’values
attributeAttach units to these arrays using MetPy’s
units
class
Perform these three steps¶
wspd = nysm_data['max_wind_speed_prop [m/s]'].values * units['m/s']
drct = nysm_data['wind_direction_prop [degrees]'].values * units['degree']
Examine these two units aware Series
wspd
Magnitude | [0.6 1.1 3.4 2.6 3.7 4.0 0.8 0.6 2.5 6.8 0.9 3.1 4.7 5.2 4.4 0.9 3.6 2.8 |
---|---|
Units | meter/second |
drct
Magnitude | [166 152 66 24 79 45 120 326 131 93 254 136 72 120 67 57 59 83 |
---|---|
Units | degree |
Convert wind speed from m/s to knots
wspk = wspd.to('knots')
wspk
Magnitude | [1.1663066954643628 2.1382289416846656 6.609071274298056 5.053995680345573 |
---|---|
Units | knot |
Perform the vector decomposition¶
u, v = wind_components(wspk, drct)
Take a look at one of the output components:
u
Magnitude | [-0.28215512661732306 -1.003837682846721 -6.037687041871186 |
---|---|
Units | knot |
# Write your code here
#%load /spare11/atm350/common/mar12/metpy1.py
Now, let’s plot several of the meteorological values on a map. We will use Matplotlib and Cartopy, as well as MetPy’s StationPlot
method.
Create units-aware objects for relative humidity and station pressure
rh = nysm_data['relative_humidity [percent]'].values * units('percent')
pres = nysm_data['station_pressure [mbar]'].values * units('mbar')
Plot the map, centered over NYS, with add some geographic features, and the mesonet data.¶
Determine the current time, for use in the plot’s title and a version to be saved to disk.
timeString = nysm_data['time'][0]
timeObj = datetime.strptime(timeString,"%Y-%m-%d %H:%M:%S")
titleString = datetime.strftime(timeObj,"%B %d %Y, %H%M UTC")
figString = datetime.strftime(timeObj,"%Y%m%d_%H%M")
Previously, we used the ax.scatter
and ax.text
methods to plot markers for the stations and their site id’s. The latter method does not provide an intuitive means to orient several text stings relative to a central point as we typically do for a meteorological surface station plot. Let’s take advantage of the Metpy
package, and its StationPlot method!¶
Be patient: this may take a minute or so to plot, if you chose the highest resolution for the shapefiles!¶
Specify two resolutions: one for the Natural Earth shapefiles, and the other for MetPy’s US County shapefiles.¶
res = '10m'
resCounty = '5m'
# Set the domain for defining the plot region.
latN = 45.2
latS = 40.2
lonW = -80.0
lonE = -72.0
cLat = (latN + latS)/2
cLon = (lonW + lonE )/2
proj = ccrs.LambertConformal(central_longitude=cLon, central_latitude=cLat)
fig = plt.figure(figsize=(18,12),dpi=150) # Increase the dots per inch from default 100 to make plot easier to read
ax = fig.add_subplot(1,1,1,projection=proj)
ax.set_extent ([lonW,lonE,latS,latN])
ax.set_facecolor(cfeature.COLORS['water'])
ax.add_feature (cfeature.STATES.with_scale(res))
ax.add_feature (cfeature.RIVERS.with_scale(res))
ax.add_feature (cfeature.LAND.with_scale(res))
ax.add_feature (cfeature.COASTLINE.with_scale(res))
ax.add_feature (cfeature.LAKES.with_scale(res))
ax.add_feature (cfeature.STATES.with_scale(res))
ax.add_feature(USCOUNTIES.with_scale(resCounty), linewidth=0.8, edgecolor='darkgray')
# Create a station plot pointing to an Axes to draw on as well as the location of points
stationplot = StationPlot(ax, lons, lats, transform=ccrs.PlateCarree(),
fontsize=8)
stationplot.plot_parameter('NW', tmpf, color='red')
stationplot.plot_parameter('SW', rh, color='green')
stationplot.plot_parameter('NE', pres, color='purple')
stationplot.plot_barb(u, v,zorder=2) # zorder value set so wind barbs will display over lake features
plotTitle = f'NYSM Temperature(°F), RH (%), Station Pressure (hPa), Peak 5-min Wind (kts), {titleString}'
ax.set_title (plotTitle);
---------------------------------------------------------------------------
NameError Traceback (most recent call last)
Cell In[17], line 28
24 # Create a station plot pointing to an Axes to draw on as well as the location of points
25 stationplot = StationPlot(ax, lons, lats, transform=ccrs.PlateCarree(),
26 fontsize=8)
---> 28 stationplot.plot_parameter('NW', tmpf, color='red')
29 stationplot.plot_parameter('SW', rh, color='green')
30 stationplot.plot_parameter('NE', pres, color='purple')
NameError: name 'tmpf' is not defined
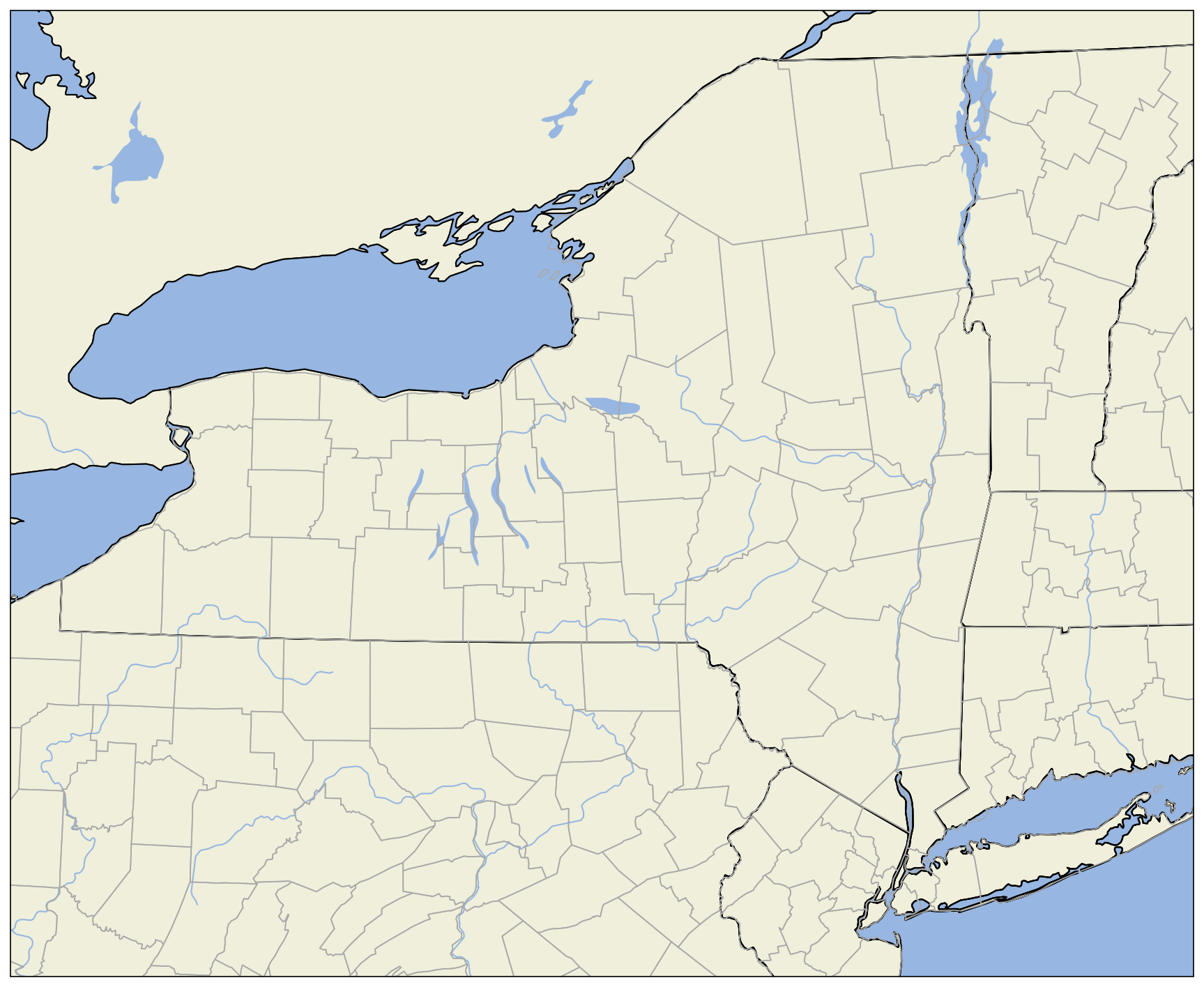
What if we wanted to plot sea-level pressure (SLP) instead of station pressure? In this case, we can apply what’s called a reduction to sea-level pressure formula. This formula requires station elevation (accounting for sensor height) in meters, temperature in Kelvin, and station pressure in hectopascals. We assume each NYSM station has its sensor height .5 meters above ground level.
pres.m
array([ 954.03, 954.6 , 980.01, 1004.79, 959.11, 996.04, 963.95,
967.12, 951.81, 1011.28, 985.43, 994.17, 992.57, 1008.57,
955.76, 1002.77, 990.27, 947.54, 1000.81, 996.25, 1004.09,
1011.82, 978.33, 963.89, 948.08, 990.11, 959.92, 977.72,
944.08, 964.51, 991.19, 985. , 965.58, 997. , 941.23,
977.35, 1000.35, 967.51, 967.71, 978.45, 990.01, 974. ,
982.52, 973.71, 1012.14, 991.88, 983. , 954.11, 1005.82,
984.31, 967.17, 942.64, 1001.76, 954.93, 956.64, 929.95,
989.9 , 993.06, 958.8 , 989.6 , 997.84, 1007.97, 957.17,
1006.17, 988.48, 1003.99, 993.33, 971.82, nan, 961.94,
958.04, 982.67, 952.74, 959.44, 1001.3 , 973.67, 975. ,
1001.96, 987.16, 960.92, 983.06, 998.85, 954.03, 1000.4 ,
1008.83, 960.25, 952.7 , 969.19, 1008.33, 949.46, 991.18,
981.1 , 971.91, 1005. , 1004.05, 1013.58, 966.58, 972.53,
993.98, 1016.35, 992.74, 962.82, 1010.68, 982.18, 1011.19,
994.34, 934.3 , 1008.48, 968.97, 957.26, 970.05, 1003.17,
1002.64, 949.69, 1014.8 , 950.03, 995.19, 995.08, 965.06,
1004.2 , 990.51, 946.02, 962.93, 1013.85, 998.47, 991.56])
elev = nysm_data['elevation']
sensorHeight = .5
# Reduce station pressure to SLP. Source: https://www.sandhurstweather.org.uk/barometric.pdf
slp = pres.m/np.exp(-1*(elev+sensorHeight)/((tmpc.m + 273.15) * 29.263))
---------------------------------------------------------------------------
NameError Traceback (most recent call last)
Cell In[19], line 4
2 sensorHeight = .5
3 # Reduce station pressure to SLP. Source: https://www.sandhurstweather.org.uk/barometric.pdf
----> 4 slp = pres.m/np.exp(-1*(elev+sensorHeight)/((tmpc.m + 273.15) * 29.263))
NameError: name 'tmpc' is not defined
slp
---------------------------------------------------------------------------
NameError Traceback (most recent call last)
Cell In[20], line 1
----> 1 slp
NameError: name 'slp' is not defined
Make a new map, substituting SLP for station pressure. We will also use the convention of the three least-significant digits to represent SLP in hectopascals.
fig = plt.figure(figsize=(18,12),dpi=150) # Increase the dots per inch from default 100 to make plot easier to read
ax = fig.add_subplot(1,1,1,projection=proj)
ax.set_extent ([lonW,lonE,latS,latN])
ax.set_facecolor(cfeature.COLORS['water'])
ax.add_feature (cfeature.STATES.with_scale(res))
ax.add_feature (cfeature.RIVERS.with_scale(res))
ax.add_feature (cfeature.LAND.with_scale(res))
ax.add_feature (cfeature.COASTLINE.with_scale(res))
ax.add_feature (cfeature.LAKES.with_scale(res))
ax.add_feature (cfeature.STATES.with_scale(res))
ax.add_feature(USCOUNTIES.with_scale(resCounty), linewidth=0.8, edgecolor='darkgray')
stationplot = StationPlot(ax, lons, lats, transform=ccrs.PlateCarree(),
fontsize=8)
stationplot.plot_parameter('NW', tmpf, color='red')
stationplot.plot_parameter('SW', rh, color='green')
# A more complex example uses a custom formatter to control how the sea-level pressure
# values are plotted. This uses the standard trailing 3-digits of the pressure value
# in tenths of millibars.
stationplot.plot_parameter('NE', slp, color='purple', formatter=lambda v: format(10 * v, '.0f')[-3:])
stationplot.plot_barb(u, v,zorder=2)
plotTitle = f'NYSM Temperature(°F), RH (%), SLP(hPa), Peak 5-min Wind (kts), {titleString}'
ax.set_title (plotTitle);
---------------------------------------------------------------------------
NameError Traceback (most recent call last)
Cell In[21], line 17
12 ax.add_feature(USCOUNTIES.with_scale(resCounty), linewidth=0.8, edgecolor='darkgray')
14 stationplot = StationPlot(ax, lons, lats, transform=ccrs.PlateCarree(),
15 fontsize=8)
---> 17 stationplot.plot_parameter('NW', tmpf, color='red')
18 stationplot.plot_parameter('SW', rh, color='green')
19 # A more complex example uses a custom formatter to control how the sea-level pressure
20 # values are plotted. This uses the standard trailing 3-digits of the pressure value
21 # in tenths of millibars.
NameError: name 'tmpf' is not defined
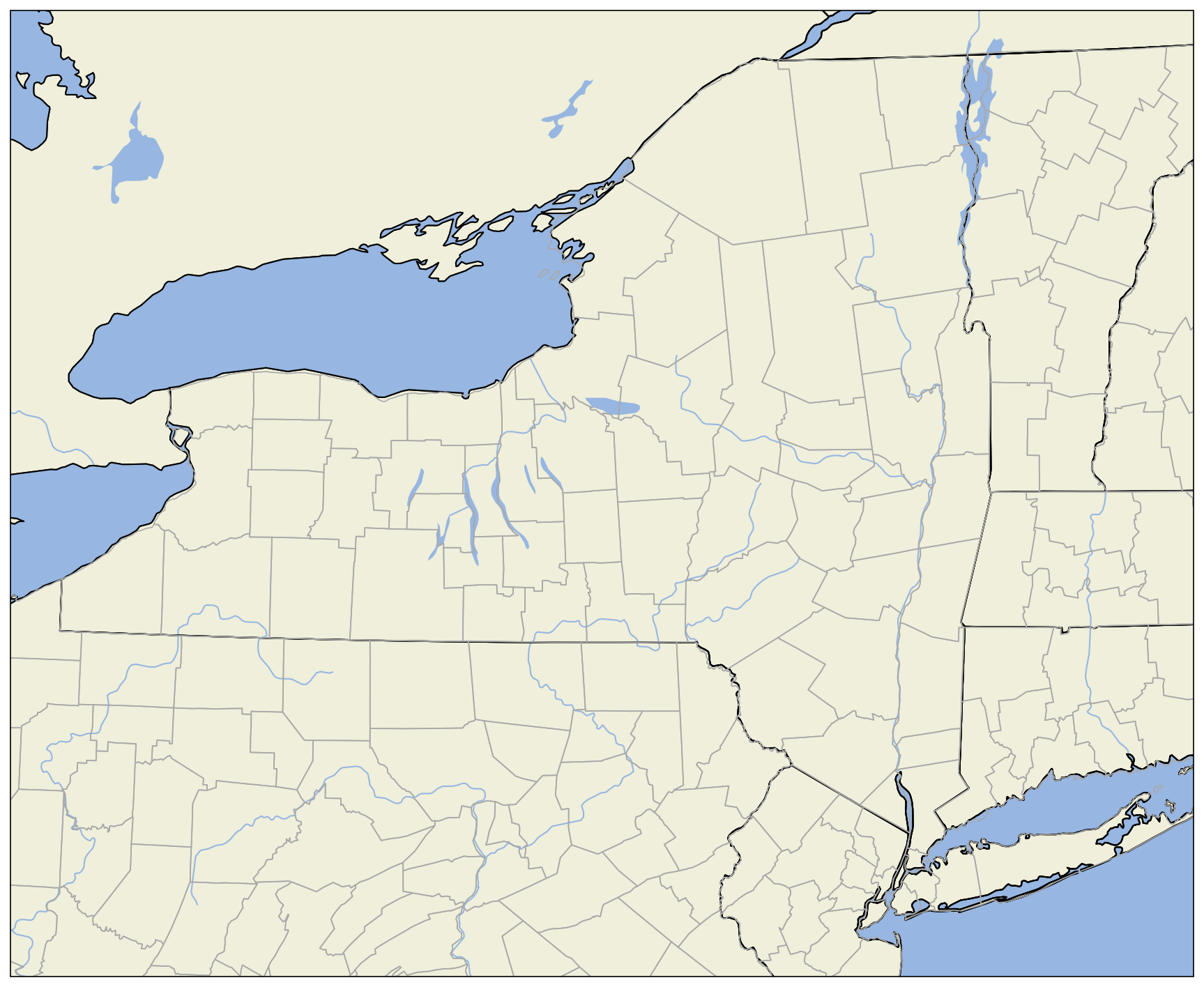
One last thing to do … plot dewpoint instead of RH. MetPy’s dewpoint_from_relative_humidity takes care of this!
dwpc = dewpoint_from_relative_humidity(tmpf, rh)
---------------------------------------------------------------------------
NameError Traceback (most recent call last)
Cell In[22], line 1
----> 1 dwpc = dewpoint_from_relative_humidity(tmpf, rh)
NameError: name 'tmpf' is not defined
dwpc
---------------------------------------------------------------------------
NameError Traceback (most recent call last)
Cell In[23], line 1
----> 1 dwpc
NameError: name 'dwpc' is not defined
The dewpoint is returned in units of degrees Celsius, so convert to Fahrenheit.
dwpf = dwpc.to('degF')
---------------------------------------------------------------------------
NameError Traceback (most recent call last)
Cell In[24], line 1
----> 1 dwpf = dwpc.to('degF')
NameError: name 'dwpc' is not defined
Plot the map
res = '10m'
fig = plt.figure(figsize=(18,12),dpi=150) # Increase the dots per inch from default 100 to make plot easier to read
ax = fig.add_subplot(1,1,1,projection=proj)
ax.set_extent ([lonW,lonE,latS,latN])
ax.set_facecolor(cfeature.COLORS['water'])
ax.add_feature(cfeature.STATES.with_scale(res))
ax.add_feature(cfeature.RIVERS.with_scale(res))
ax.add_feature (cfeature.LAND.with_scale(res))
ax.add_feature(cfeature.COASTLINE.with_scale(res))
ax.add_feature (cfeature.LAKES.with_scale(res))
ax.add_feature (cfeature.STATES.with_scale(res))
ax.add_feature(USCOUNTIES.with_scale(resCounty), linewidth=0.8, edgecolor='darkgray')
stationplot = StationPlot(ax, lons, lats, transform=ccrs.PlateCarree(),
fontsize=8)
stationplot.plot_parameter('NW', tmpf, color='red')
stationplot.plot_parameter('SW', dwpf, color='green')
# A more complex example uses a custom formatter to control how the sea-level pressure
# values are plotted. This uses the standard trailing 3-digits of the pressure value
# in tenths of millibars.
stationplot.plot_parameter('NE', slp, color='purple', formatter=lambda v: format(10 * v, '.0f')[-3:])
stationplot.plot_barb(u, v,zorder=2)
plotTitle = f'NYSM Temperature and Dewpoint(°F), SLP (hPa), Peak 5-min Wind (kts), {titleString}'
ax.set_title (plotTitle);
---------------------------------------------------------------------------
NameError Traceback (most recent call last)
Cell In[25], line 18
13 ax.add_feature(USCOUNTIES.with_scale(resCounty), linewidth=0.8, edgecolor='darkgray')
15 stationplot = StationPlot(ax, lons, lats, transform=ccrs.PlateCarree(),
16 fontsize=8)
---> 18 stationplot.plot_parameter('NW', tmpf, color='red')
19 stationplot.plot_parameter('SW', dwpf, color='green')
20 # A more complex example uses a custom formatter to control how the sea-level pressure
21 # values are plotted. This uses the standard trailing 3-digits of the pressure value
22 # in tenths of millibars.
NameError: name 'tmpf' is not defined
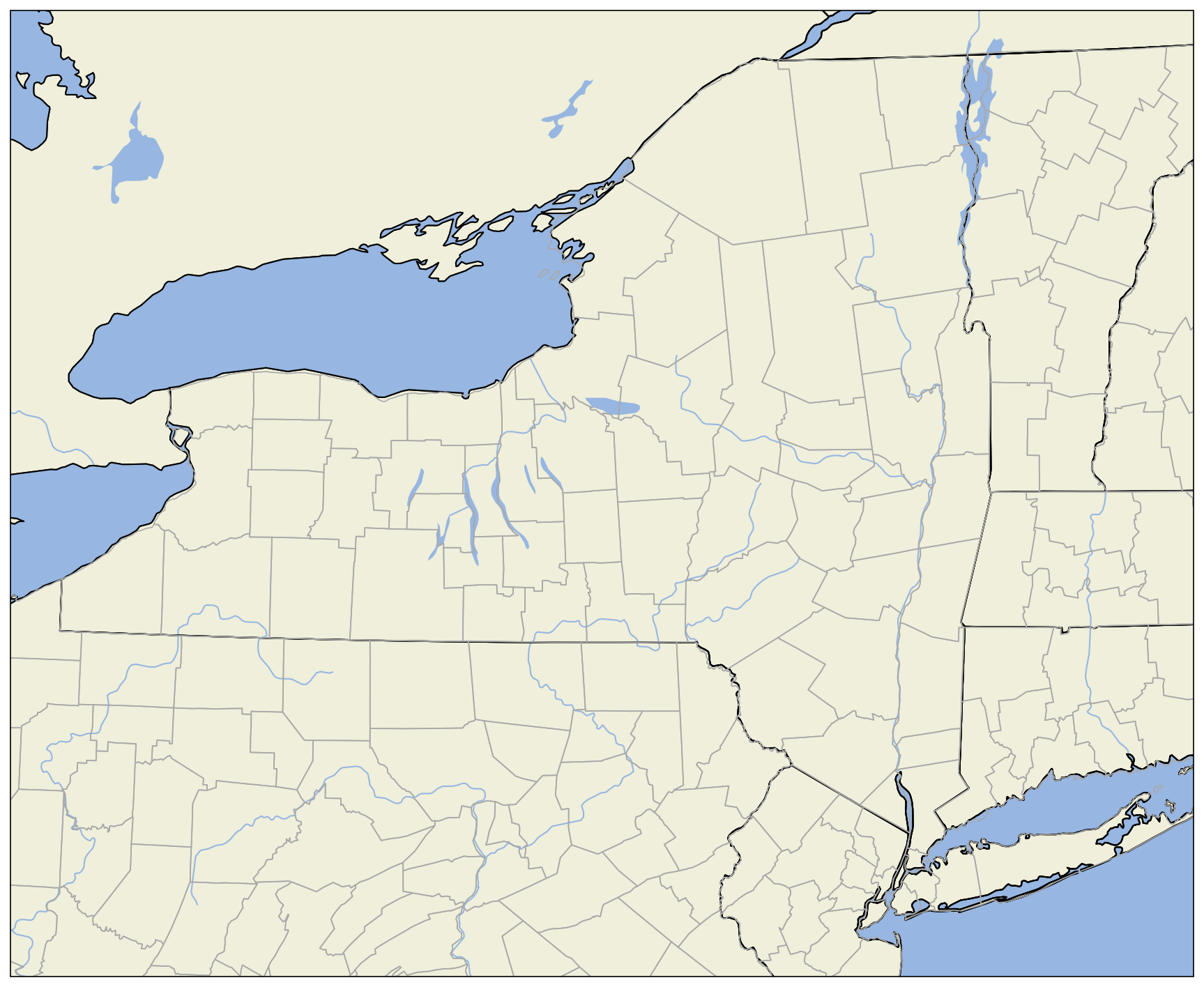
Save the plot to the current directory.
figName = f'NYSM_{figString}.png'
figName
'NYSM_20240430_0200.png'
fig.savefig(figName)
Summary¶
The MetPy library provides methods to assign physical units to numerical arrays and perform units-aware calculations
MetPy’s
StationPlot
method offers a customized use of Matplotlib’s Pyplot library to plot several meteorologically-relevant parameters centered about several geo-referenced points.
What’s Next?¶
In the next notebook, we will plot METAR observations from sites across the world, using a different access method to retrieve the data.