Xarray 5: Time Series
Contents

Xarray 5: Time Series¶
Overview¶
Work with an ERA-5
Dataset
hosted on NCAR’s Research Data ArchiveSubset the Dataset along its dimensions
Perform diagnostic calculations and unit conversions
Perform Split-Apply-Combine
Create and refine a time series plot of minimum SLP and maximum windspeed over the subsetted region
Imports¶
import xarray as xr
import pandas as pd
import numpy as np
from datetime import datetime as dt
from metpy.units import units
import metpy.calc as mpcalc
import cartopy.crs as ccrs
import cartopy.feature as cfeature
import matplotlib.pyplot as plt
from matplotlib.dates import DateFormatter, AutoDateLocator,HourLocator,DayLocator,MonthLocator
from matplotlib.ticker import (MultipleLocator, AutoMinorLocator)
import requests
Work with an ERA-5 Dataset hosted on NCAR’s Remote Data Archive¶
Create OpenDAP URLs pointing to the variables as they are stored in RDA’s THREDDS server
ds_urlu = 'https://thredds.rda.ucar.edu/thredds/dodsC/files/g/ds633.0/e5.oper.an.sfc/201210/e5.oper.an.sfc.128_165_10u.ll025sc.2012100100_2012103123.nc'
ds_urlv = 'https://thredds.rda.ucar.edu/thredds/dodsC/files/g/ds633.0/e5.oper.an.sfc/201210/e5.oper.an.sfc.128_166_10v.ll025sc.2012100100_2012103123.nc'
ds_urlp = 'https://thredds.rda.ucar.edu/thredds/dodsC/files/g/ds633.0/e5.oper.an.sfc/201210/e5.oper.an.sfc.128_151_msl.ll025sc.2012100100_2012103123.nc'
Now open the three files as separate Dataset
s
dsSLP = xr.open_dataset(ds_urlp)
dsU10 = xr.open_dataset(ds_urlu)
dsV10 = xr.open_dataset(ds_urlv)
Examine one of the Dataset
s.
dsU10
<xarray.Dataset> Dimensions: (latitude: 721, longitude: 1440, time: 744) Coordinates: * latitude (latitude) float64 90.0 89.75 89.5 89.25 ... -89.5 -89.75 -90.0 * longitude (longitude) float64 0.0 0.25 0.5 0.75 ... 359.0 359.2 359.5 359.8 * time (time) datetime64[ns] 2012-10-01 ... 2012-10-31T23:00:00 Data variables: utc_date (time) int32 ... VAR_10U (time, latitude, longitude) float32 ... Attributes: _NCProperties: version=1|netcdflibversion=4.6.1|hdf5lib... DATA_SOURCE: ECMWF: https://cds.climate.copernicus.eu... NETCDF_CONVERSION: CISL RDA: Conversion from ECMWF GRIB1 da... NETCDF_VERSION: 4.6.1 CONVERSION_PLATFORM: Linux casper21 3.10.0-693.21.1.el7.x86_6... CONVERSION_DATE: Mon Aug 26 16:10:34 MDT 2019 Conventions: CF-1.6 NETCDF_COMPRESSION: NCO: Precision-preserving compression to... history: Mon Aug 26 16:11:11 2019: ncks -4 --ppc ... NCO: netCDF Operators version 4.7.4 (http://n... DODS_EXTRA.Unlimited_Dimension: time
Create DataArray
objects for each of the three variables in the Dataset
s.
slp = dsSLP['MSL']
u10 = dsU10['VAR_10U']
v10 = dsV10['VAR_10V']
Subset the Dataset along its dimensions.¶
As we did before, we will perform temporal and areal subsetting.
Take advantage of Pandas’ date/time methods. In this case, we will use Pandas date_range.¶
Let’s define a function whose output we will use to label our plotted time series.
timeRange = pd.date_range(start="2012-10-27 00:00",end="2012-10-31 00:00",freq='1H')
timeRange
DatetimeIndex(['2012-10-27 00:00:00', '2012-10-27 01:00:00',
'2012-10-27 02:00:00', '2012-10-27 03:00:00',
'2012-10-27 04:00:00', '2012-10-27 05:00:00',
'2012-10-27 06:00:00', '2012-10-27 07:00:00',
'2012-10-27 08:00:00', '2012-10-27 09:00:00',
'2012-10-27 10:00:00', '2012-10-27 11:00:00',
'2012-10-27 12:00:00', '2012-10-27 13:00:00',
'2012-10-27 14:00:00', '2012-10-27 15:00:00',
'2012-10-27 16:00:00', '2012-10-27 17:00:00',
'2012-10-27 18:00:00', '2012-10-27 19:00:00',
'2012-10-27 20:00:00', '2012-10-27 21:00:00',
'2012-10-27 22:00:00', '2012-10-27 23:00:00',
'2012-10-28 00:00:00', '2012-10-28 01:00:00',
'2012-10-28 02:00:00', '2012-10-28 03:00:00',
'2012-10-28 04:00:00', '2012-10-28 05:00:00',
'2012-10-28 06:00:00', '2012-10-28 07:00:00',
'2012-10-28 08:00:00', '2012-10-28 09:00:00',
'2012-10-28 10:00:00', '2012-10-28 11:00:00',
'2012-10-28 12:00:00', '2012-10-28 13:00:00',
'2012-10-28 14:00:00', '2012-10-28 15:00:00',
'2012-10-28 16:00:00', '2012-10-28 17:00:00',
'2012-10-28 18:00:00', '2012-10-28 19:00:00',
'2012-10-28 20:00:00', '2012-10-28 21:00:00',
'2012-10-28 22:00:00', '2012-10-28 23:00:00',
'2012-10-29 00:00:00', '2012-10-29 01:00:00',
'2012-10-29 02:00:00', '2012-10-29 03:00:00',
'2012-10-29 04:00:00', '2012-10-29 05:00:00',
'2012-10-29 06:00:00', '2012-10-29 07:00:00',
'2012-10-29 08:00:00', '2012-10-29 09:00:00',
'2012-10-29 10:00:00', '2012-10-29 11:00:00',
'2012-10-29 12:00:00', '2012-10-29 13:00:00',
'2012-10-29 14:00:00', '2012-10-29 15:00:00',
'2012-10-29 16:00:00', '2012-10-29 17:00:00',
'2012-10-29 18:00:00', '2012-10-29 19:00:00',
'2012-10-29 20:00:00', '2012-10-29 21:00:00',
'2012-10-29 22:00:00', '2012-10-29 23:00:00',
'2012-10-30 00:00:00', '2012-10-30 01:00:00',
'2012-10-30 02:00:00', '2012-10-30 03:00:00',
'2012-10-30 04:00:00', '2012-10-30 05:00:00',
'2012-10-30 06:00:00', '2012-10-30 07:00:00',
'2012-10-30 08:00:00', '2012-10-30 09:00:00',
'2012-10-30 10:00:00', '2012-10-30 11:00:00',
'2012-10-30 12:00:00', '2012-10-30 13:00:00',
'2012-10-30 14:00:00', '2012-10-30 15:00:00',
'2012-10-30 16:00:00', '2012-10-30 17:00:00',
'2012-10-30 18:00:00', '2012-10-30 19:00:00',
'2012-10-30 20:00:00', '2012-10-30 21:00:00',
'2012-10-30 22:00:00', '2012-10-30 23:00:00',
'2012-10-31 00:00:00'],
dtype='datetime64[ns]', freq='H')
def areaBox(lonW,lonE,latS,latN):
'''
Returns a string containing the bounds of the selected region
'''
outputStr = ''
for val in [lonW, lonE]:
if (val < 0):
val *= -1
valStr = str(val) + 'W '
else:
valStr = str(val) + 'E '
outputStr = outputStr + valStr
for val in [latS, latN]:
if (val< 0):
val *= -1
valStr = str(val) + 'S '
else:
valStr = str(val) + 'N '
outputStr = outputStr + valStr
return outputStr
# Areal extent
lonW = -100
lonE = -60
latS = 20
latN = 50
domainStr = areaBox(lonW,lonE,latS,latN)
latRange = np.arange(latS-5,latN+5,.25) # expand the data range a bit beyond the plot range
lonRange = np.arange((lonW-5+360),(lonE+5+360),.25) # Need to match longitude values to those of the coordinate variable
# Vertical level specificaton
vlevel = 10
levelStr = str(vlevel)
# Data variable selection
SLP = dsSLP['MSL'].sel(time=timeRange,latitude=latRange,longitude=lonRange)
U10 = dsU10['VAR_10U'].sel(time=timeRange,latitude=latRange,longitude=lonRange)
V10 = dsV10['VAR_10V'].sel(time=timeRange,latitude=latRange,longitude=lonRange)
Examine a couple of the DataArrays following our subsetting
SLP
<xarray.DataArray 'MSL' (time: 97, latitude: 160, longitude: 200)> [3104000 values with dtype=float32] Coordinates: * latitude (latitude) float64 15.0 15.25 15.5 15.75 ... 54.25 54.5 54.75 * longitude (longitude) float64 255.0 255.2 255.5 255.8 ... 304.2 304.5 304.8 * time (time) datetime64[ns] 2012-10-27 ... 2012-10-31 Attributes: (12/15) long_name: Mean sea level pressure short_name: msl units: Pa original_format: WMO GRIB 1 with ECMWF local table ecmwf_local_table: 128 ecmwf_parameter: 151 ... ... rda_dataset: ds633.0 rda_dataset_url: https:/rda.ucar.edu/datasets/ds633.0/ rda_dataset_doi: DOI: 10.5065/BH6N-5N20 rda_dataset_group: ERA5 atmospheric surface analysis [netCDF4] number_of_significant_digits: 7 _ChunkSizes: [ 27 139 277]
U10
<xarray.DataArray 'VAR_10U' (time: 97, latitude: 160, longitude: 200)> [3104000 values with dtype=float32] Coordinates: * latitude (latitude) float64 15.0 15.25 15.5 15.75 ... 54.25 54.5 54.75 * longitude (longitude) float64 255.0 255.2 255.5 255.8 ... 304.2 304.5 304.8 * time (time) datetime64[ns] 2012-10-27 ... 2012-10-31 Attributes: (12/15) long_name: 10 metre U wind component short_name: 10u units: m s**-1 original_format: WMO GRIB 1 with ECMWF local table ecmwf_local_table: 128 ecmwf_parameter: 165 ... ... rda_dataset: ds633.0 rda_dataset_url: https:/rda.ucar.edu/datasets/ds633.0/ rda_dataset_doi: DOI: 10.5065/BH6N-5N20 rda_dataset_group: ERA5 atmospheric surface analysis [netCDF4] number_of_significant_digits: 7 _ChunkSizes: [ 27 139 277]
Xarray has Split-Apply-Combine functionality similar to Pandas. In this next cell, we are grouping by date/time and then calculating the minimum value of SLP over the other two dimensions.
SLP.min(dim=['latitude','longitude'])
<xarray.DataArray 'MSL' (time: 97)> array([97424.19 , 97386.19 , 97329.19 , 97283.56 , 97188.625, 97090.5 , 96980.06 , 96884.75 , 96826.31 , 96794.69 , 96577.31 , 96662.875, 96732.56 , 96743.56 , 96804.69 , 96821.19 , 96793.81 , 96735.31 , 96649.56 , 96590.44 , 96545.125, 96529.44 , 96340.31 , 96368.94 , 96400.81 , 96408.81 , 96400.31 , 96402.25 , 96376. , 96360.31 , 96353.75 , 96312.75 , 96288.31 , 96257.19 , 96062.44 , 96102.56 , 96251.69 , 96306.31 , 96344.69 , 96323.75 , 96257.19 , 96140.625, 96012.69 , 95967.94 , 95969.94 , 95922.5 , 95758.81 , 95805.875, 95845.44 , 95862.81 , 95877.5 , 95845.25 , 95809.56 , 95795.94 , 95812.625, 95817.44 , 95791.875, 95702.5 , 95573.06 , 95526.875, 95537.06 , 95547.625, 95507.06 , 95456.125, 95367.5 , 95192.125, 94923.81 , 94701.875, 94506.5 , 94472.56 , 94594.625, 94716.375, 94913.69 , 95227. , 95453.25 , 95703.625, 95893.56 , 96158.81 , 96345.31 , 96559.5 , 96739.75 , 96902.69 , 97443.81 , 97620.875, 97852.81 , 98060. , 98242. , 98384.625, 98537.75 , 98662.75 , 98757.69 , 98799.69 , 98886.125, 98964.31 , 99187.69 , 99301.56 , 99346.625], dtype=float32) Coordinates: * time (time) datetime64[ns] 2012-10-27 2012-10-27T01:00:00 ... 2012-10-31
Call Xarray’s built-in plot
method to get a quick look at the time series. Note that we use the Python convention where _
is an object corresponding to the output of the previous line of code.
_.plot()
[<matplotlib.lines.Line2D at 0x14bf340a7f90>]
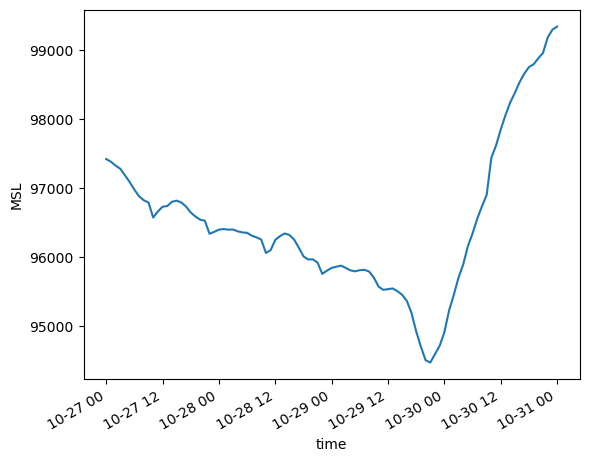
Perform unit conversions¶
Convert meters per second to knots, and Pascals to hectoPascals. Both are straightforward with MetPy’s convert_units
method.
SLP = SLP.metpy.convert_units('hPa')
U10 = U10.metpy.convert_units('kts')
V10 = V10.metpy.convert_units(‘kts’)
Calculate wind speed from the u- and v- components using MetPy’s diagnostic function, wind_speed
.
WSPD = mpcalc.wind_speed(U10,V10)
Perform Split-Apply-Combine¶
Compute the minimum SLP, and then the maximum wind speed, for all latitude and longitude points … for each time.
SLPmin = SLP.min(dim=['latitude','longitude'])
print(SLPmin)
<xarray.DataArray 'MSL' (time: 97)>
<Quantity([974.2419 973.8619 973.2919 972.83563 971.8862 970.90497 969.8006
968.8475 968.2631 967.94684 965.77313 966.6287 967.3256 967.4356
968.0469 968.21185 967.9381 967.3531 966.4956 965.90436 965.45123
965.2944 963.4031 963.68933 964.0081 964.0881 964.0031 964.02246
963.75995 963.6031 963.5375 963.1275 962.8831 962.57184 960.6243
961.0256 962.51685 963.0631 963.44684 963.2375 962.57184 961.40625
960.12683 959.6794 959.69934 959.225 957.5881 958.0587 958.45435
958.6281 958.77496 958.45245 958.0956 957.95935 958.1262 958.1744
957.9187 957.02496 955.7306 955.26874 955.3706 955.47626 955.0706
954.5612 953.675 951.9212 949.2381 947.01874 945.065 944.7256
945.9462 947.16376 949.13684 952.26996 954.5325 957.03625 958.9356
961.5881 963.4531 965.595 967.39746 969.02686 974.4381 976.20874
978.5281 980.6 982.42 983.84625 985.3775 986.6275 987.57684
987.9968 988.8612 989.6431 991.87683 993.0156 993.46625], 'hectopascal')>
Coordinates:
* time (time) datetime64[ns] 2012-10-27 2012-10-27T01:00:00 ... 2012-10-31
WSPDmax = WSPD.max(dim=['latitude','longitude'])
We’ll take a deeper dive into Xarray’s Split-Apply-Combine implementation in the next notebook.
Here, we’ve effectively reduced the data array down to one dimension (time)!
Create and refine a time series plot of minimum SLP and maximum windspeed over the subsetted region.¶
Create a meaningful title string.
tl1 = "ERA-5 maximum " + levelStr + " m windspeed (kts) and minimum SLP (hPa)"
tl2 = str('Valid within: '+ domainStr)
title_line = (tl1 + '\n' + tl2 + '\n')
Improve the look of the time-series figure by using Seaborn.
import seaborn as sns
sns.set()
Plot two y-axes; one for SLP, the other for windspeed
fig = plt.figure(figsize=(18,12))
ax1 = plt.subplot(1,1,1)
color = 'tab:red'
ax1.plot(SLP.time,SLPmin,color=color,label = 'Min SLP')
ax1.set_title (title_line,fontsize=14)
ax2 = ax1.twinx() # instantiate a second axes that shares the same x-axis
color = 'tab:blue'
ax2.plot(WSPD.time,WSPDmax,color=color,label = 'Max Wind');
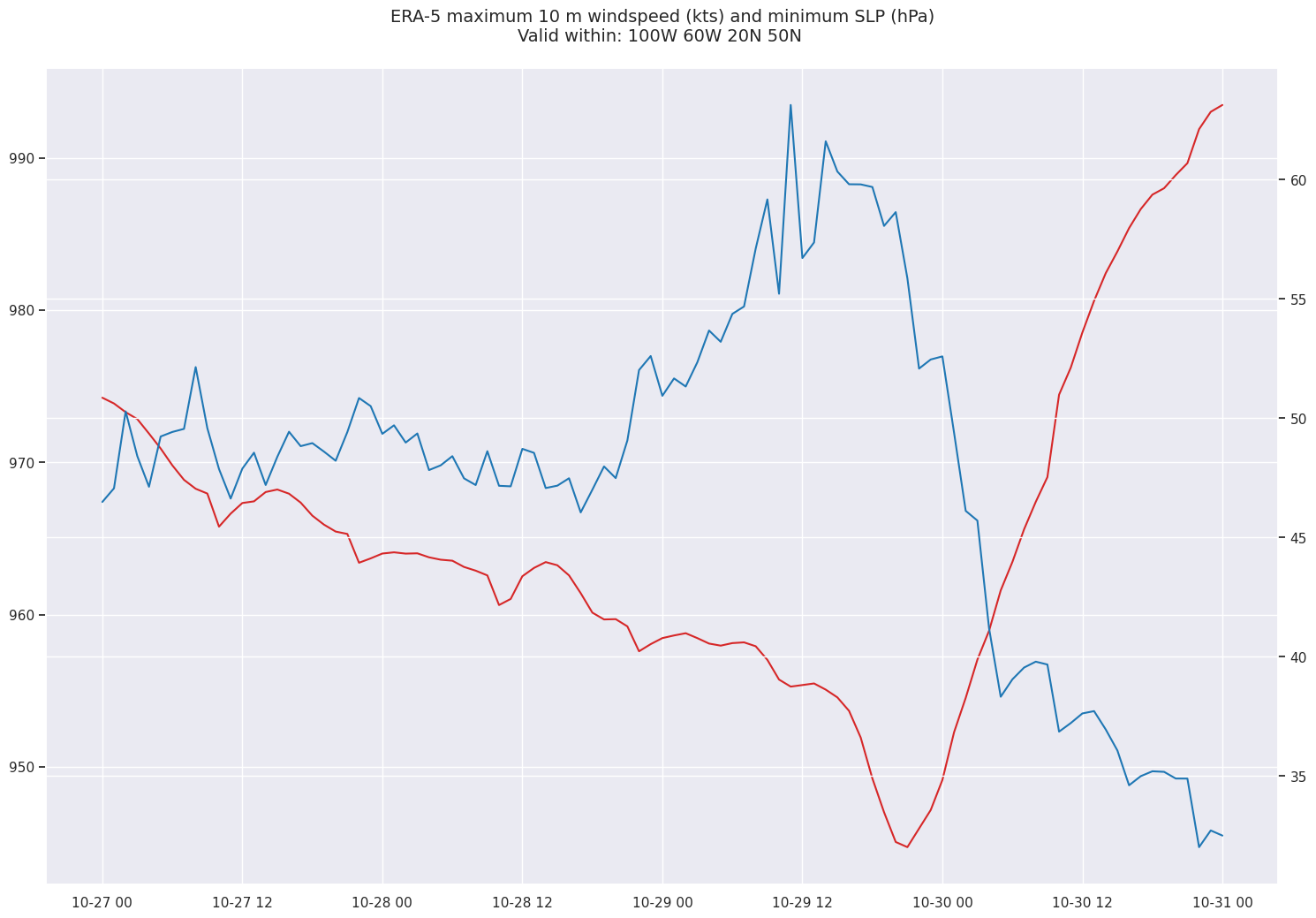
What’s the meaning of tab:
in our color
definition? It’s one of many color table specifications available in Matplotlib; in this case, tab refers to a color table derived from a commercial dataviz package, Tableau.
That’s not a bad first plot, but much can be done to improve it. Here we use several Matplotlib Axes
methods to customize the plot.¶
Note
Often the best way to learn about these methods (and others) is to browse the relevant documentation at https://matplotlib.org and play around with them. You may be the type who always wants to keep making it look better, but avoid the temptation to keep trying for perfection.
fig = plt.figure(figsize=(18,12),dpi=150)
ax1 = plt.subplot(1,1,1)
# First Axes: SLP
color = 'tab:red'
ax1.plot(SLP.time,SLPmin,color=color,label = 'Min SLP')
ax1.grid(color='black',linewidth=0.5)
ax1.xaxis.set_major_locator(HourLocator(interval=6))
ax1.xaxis.set_minor_locator(HourLocator(interval=1))
dateFmt = DateFormatter('%H')
ax1.xaxis.set_major_formatter(dateFmt)
ax1.set_xlabel('Date/Time (UTC)')
ax1.set_xlim(SLP.time[0],SLP.time[-1])
ax1.set_ylabel('SLP (hPa)',color=color, fontsize=14)
ax1.yaxis.set_minor_locator(MultipleLocator(5))
ax1.tick_params(which='minor', length=6)
ax1.tick_params(which='major',axis='y', labelcolor=color,direction='out', length=6, width=2, colors=color,
grid_color=color, grid_alpha=0.5)
ax1.tick_params(which='minor', axis = 'y', length=4, color=color)
ax1.set_title (title_line,fontsize=14)
# Second Axes: Windspeed
ax2 = ax1.twinx() # instantiate a second axes that shares the same x-axis
color = 'tab:blue'
ax2.plot(WSPD.time,WSPDmax,color='tab:blue',label = 'Max Wind')
# Do not draw grid lines (makes plot more readable)
ax2.grid(visible=None)
ax2.set_ylabel('Windspeed (kts)', color=color, fontsize=14) # we already handled the x-label with ax1
ax2.tick_params(axis='y', labelcolor=color,direction='out', length=6, width=2, colors=color,
grid_color=color, grid_alpha=0.5) # grid-related specs will be ignored since we are not plotting grid lines for this axes
fig.legend(loc='upper right',frameon=True,bbox_to_anchor=(0.9,1.1), bbox_transform=ax1.transAxes,fontsize=16,shadow=True,edgecolor='purple')
fig.autofmt_xdate(rotation=45);
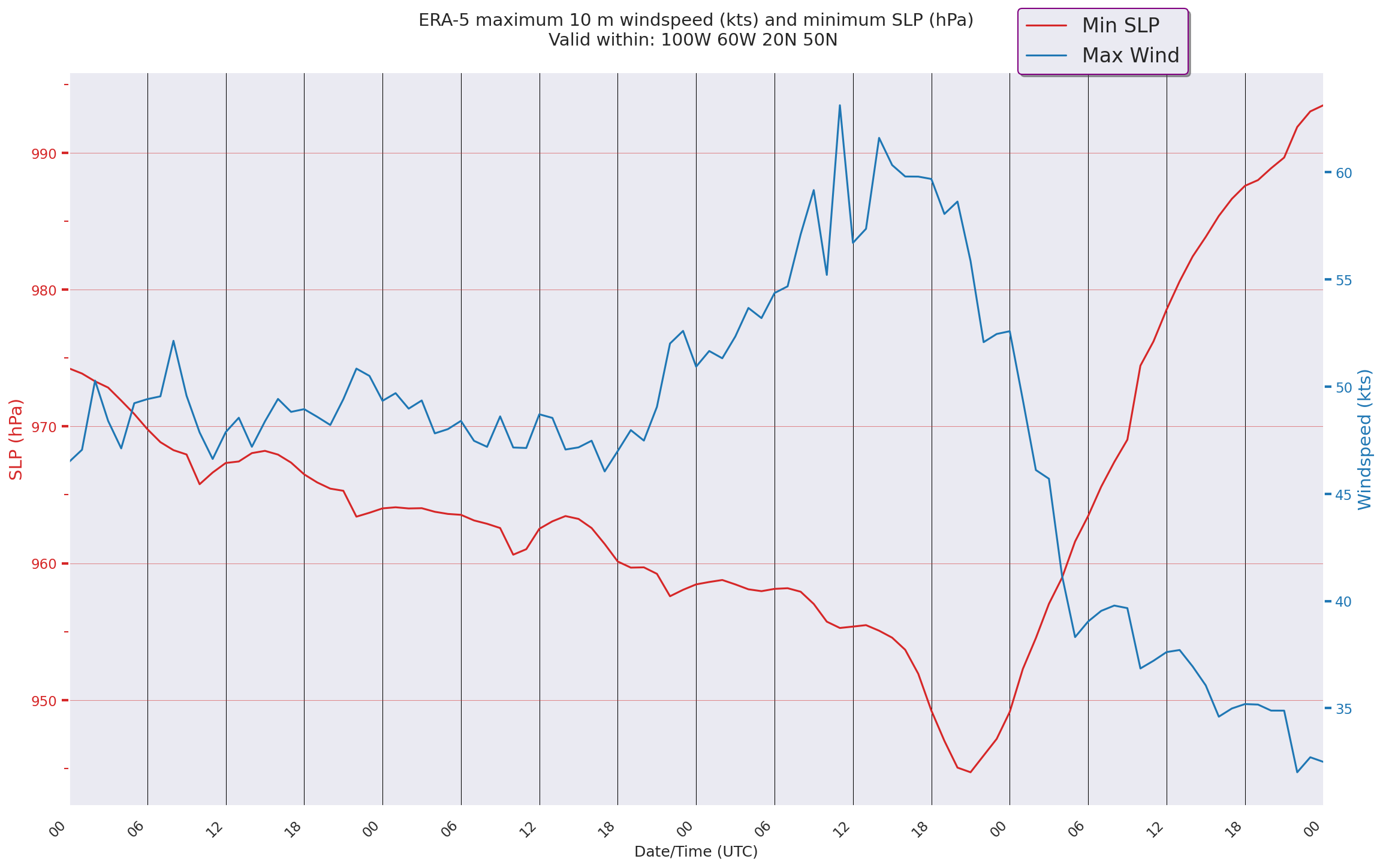
Save our figure to our current directory.
fig.savefig('ERA5_Time_Series.png')