Rasters 3: Cloud-optimized GeoTIFFs with multiple bands
Contents
Rasters 3: Cloud-optimized GeoTIFFs with multiple bands¶
Overview¶
Read in a high-resolution, cloud-optimized GeoTIFF that consists of multiple bands
Combine the bands into a single, true-color image and plot that image
Overlay the GeoTIFF on GeoAxes
Imports¶
import matplotlib.pyplot as plt
import cartopy.crs as ccrs
import cartopy.feature as cfeature
import numpy as np
import rioxarray as rxr
import rasterio
Read in a multi-band raster file in GeoTIFF format that consists of multiple bands¶
Process the GeoTIFF file, originating from Planet Labs; (see ref. 2 at end of notebook) using the rioxarray library.
rasFile = '/spare11/atm533/data/raster/la.tif'
cog = rxr.open_rasterio('/spare11/atm533/data/raster/la.tif')
cog
<xarray.DataArray (band: 4, y: 4096, x: 4096)> [67108864 values with dtype=uint8] Coordinates: * band (band) int64 1 2 3 4 * x (x) float64 -1.317e+07 -1.317e+07 ... -1.315e+07 -1.315e+07 * y (y) float64 4.011e+06 4.011e+06 ... 3.992e+06 3.992e+06 spatial_ref int64 0 Attributes: AREA_OR_POINT: Area scale_factor: 1.0 add_offset: 0.0
We can see that the raster image has 4 bands.
As we did in the 2nd Raster notebook, get the bounds of the raster file, since we need it for the extent
argument in imshow
.
left,bottom,right,top = cog.rio.bounds()
left,bottom,right,top
(-13169182.727361135,
3991847.3646168797,
-13149614.848122846,
4011415.243855167)
Get the resolution
cog.rio.resolution()
(4.77731426716, -4.77731426716)
That’s horizontal resolution of less than 5 meters!!
Get the projection info, which we can later use in Cartopy. It is EPSG 3857, aka Web Mercator.
cog.rio.crs
CRS.from_epsg(3857)
projCOG = ccrs.epsg('3857')
Plot each of the bands directly from Xarray.¶
figSize = (15,10)
for n in range(1,5):
cog.sel(band=n).plot.imshow(figsize=figSize)
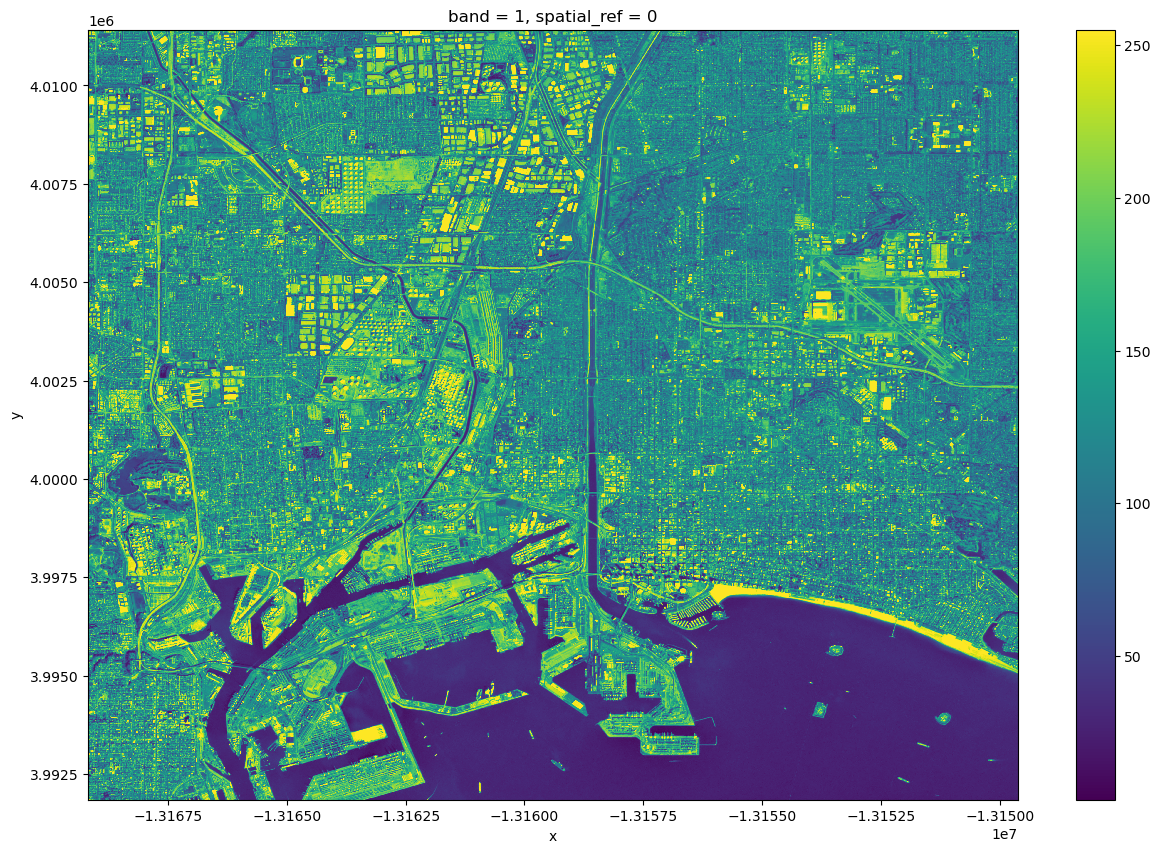
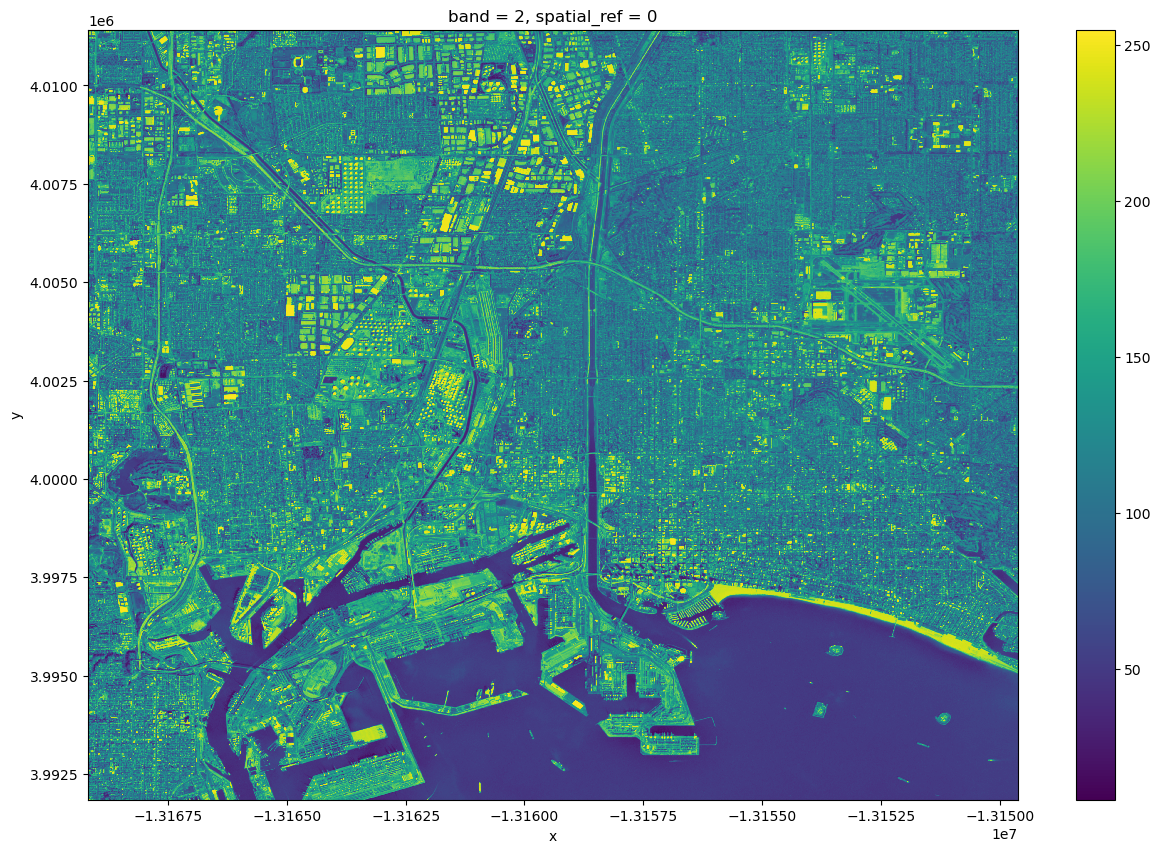
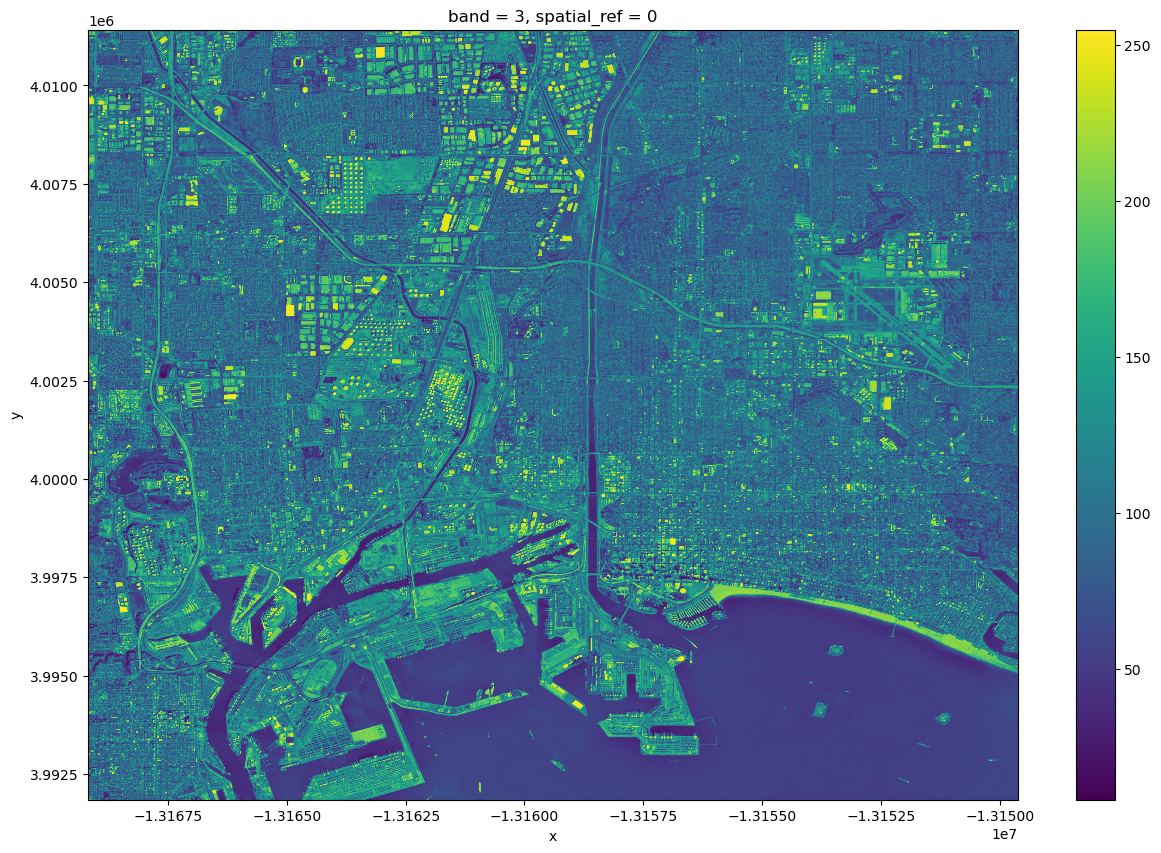
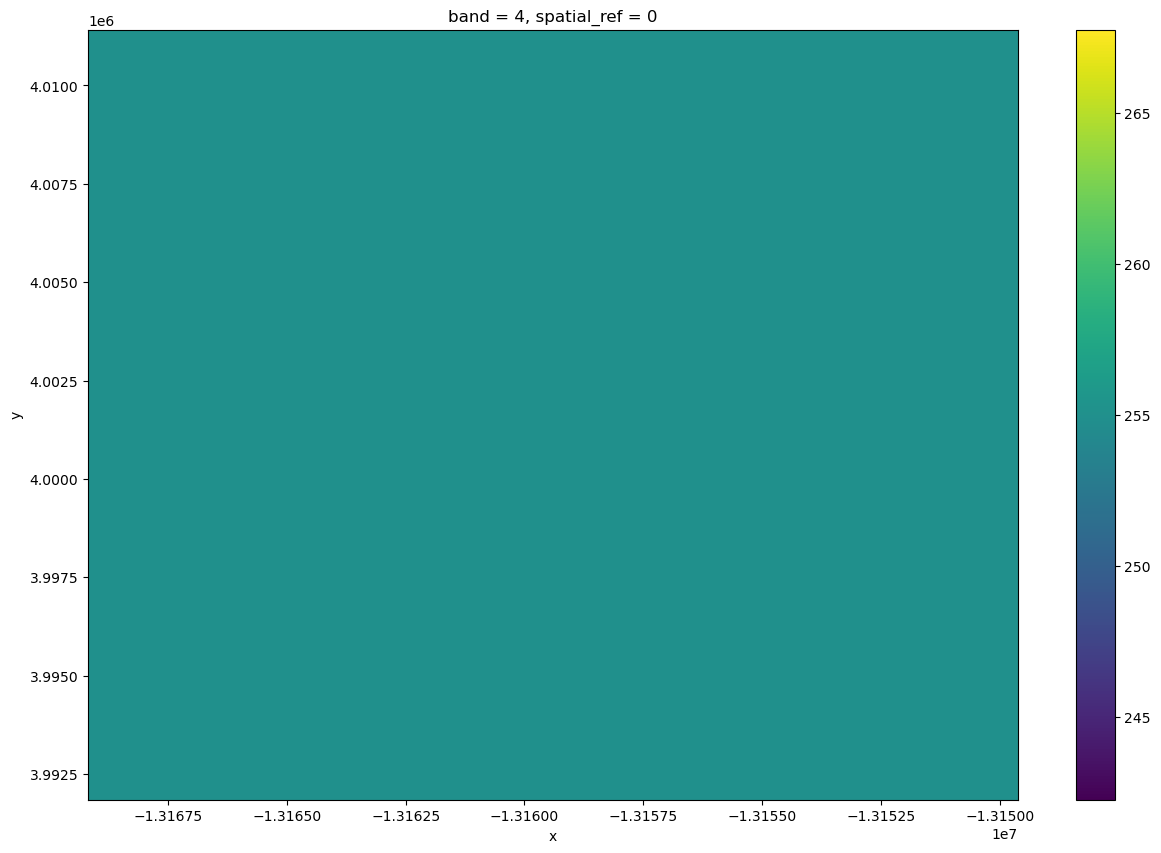
Close this Xarray DataArray as we’re done with it for now.
cog.close()
Combine the bands into a single, true-color image¶
We will now use the more general rasterio
library to deal with combining these bands into a single image.
Stack together the first 3 RGB bands to arrive at a true-color composite image (see refs. 3 and 4 at end of notebook).
rasterio
assumes that a GeoTIFF with 3 bands has a band order of red, green and blue (RGB). It interprets a 4-band image as RGB/A, where *A* denotes *alpha*, or transparency. Each of these four bands, or channels, have pixel values ranging from 0 to 255. For the three colors, the range goes from no color to saturated (full) color. For alpha, the range goes from completely transparent to completely opaque.
Rasterio’s colorinterp
method will display how it is characterizing each of these channels. The following cell demonstrates the procedure:
with rasterio.open(rasFile) as src:
# convert / read the data into a numpy array:
cog = src.read(masked= True)
red = src.read(1)
green = src.read(2)
blue = src.read(3)
print(src.colorinterp[0])
print(src.colorinterp[1])
print(src.colorinterp[2])
print(src.colorinterp[3])
3
4
5
6
cog.shape
(4, 4096, 4096)
Notice that cog
has 3 dimensions.
Now, create a function to normalize each of the three RGB bands so the ranges are from 0 to 1. We will ignore the alpha channel; for this GeoTIFF, alpha is set to 0 only for missing points, of which there are none.
def normalize(array):
array_min, array_max = array.min(), array.max()
return (array - array_min) / (array_max - array_min)
Normalize the RGB bands
red_norm = normalize(red)
green_norm = normalize(green)
blue_norm = normalize(blue)
dstack
function to create a single NumPy array that now has all three channels. This is known as a true color image.
rgb = np.dstack((red_norm, green_norm, blue_norm))
Visualize the true-color image.¶
To start, we will not create geo-referenced Matplotlib axes with Cartopy … we’ll do that shortly.
fig = plt.figure(figsize=(18,12))
plt.imshow(rgb);
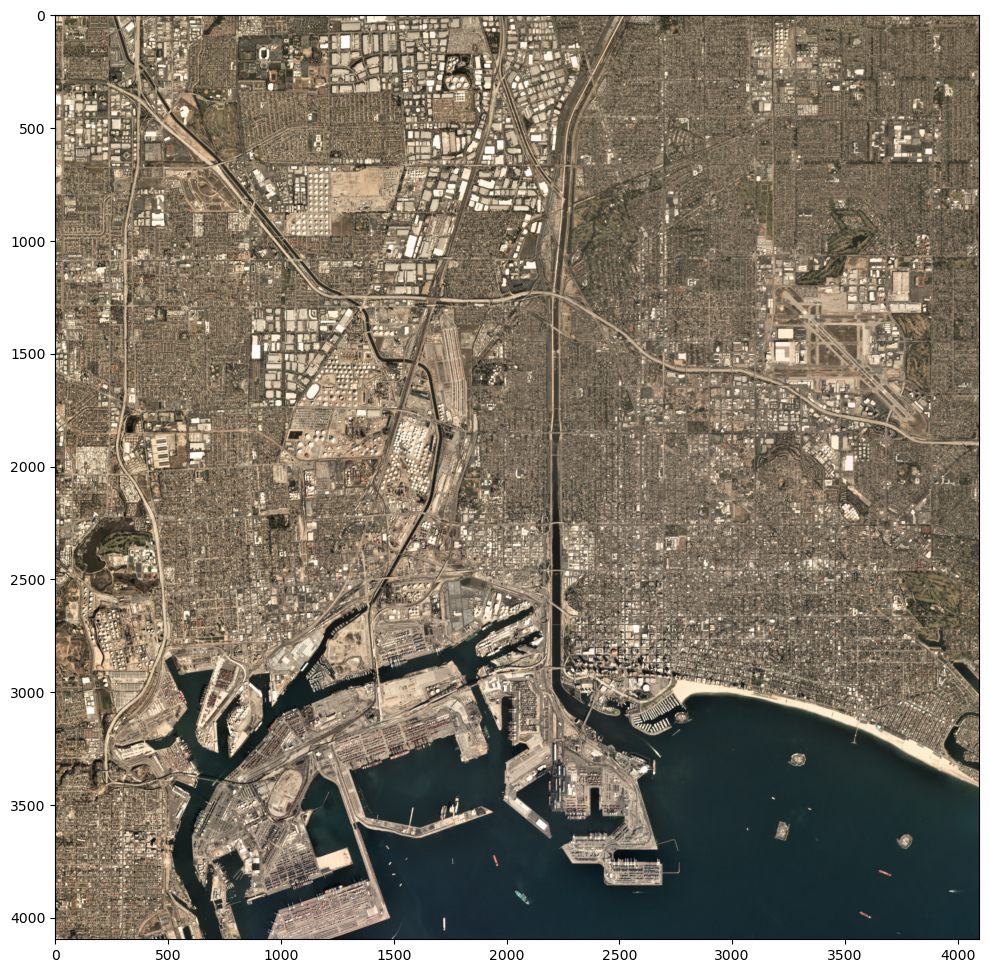
Overlay the GeoTIFF on GeoAxes¶
Now, let’s plot this image on a set of GeoAxes
. We’ll include the NaturalEarth high-res coastline shapefile which will help show how much of the plotted area is encompassed by the true-color image.
res = '10m'
fig = plt.figure(figsize=(18,12))
ax = plt.subplot(1,1,1,projection=ccrs.PlateCarree())
ax.set_extent((-118.4,-118.05,33.65,33.95),crs=ccrs.PlateCarree())
ax.gridlines(draw_labels=True,
linewidth=2, color='gray', alpha=0.5, linestyle='--')
ax.add_feature(cfeature.COASTLINE.with_scale(res))
im = ax.imshow(rgb,extent=[left, right, bottom, top],transform=projCOG)
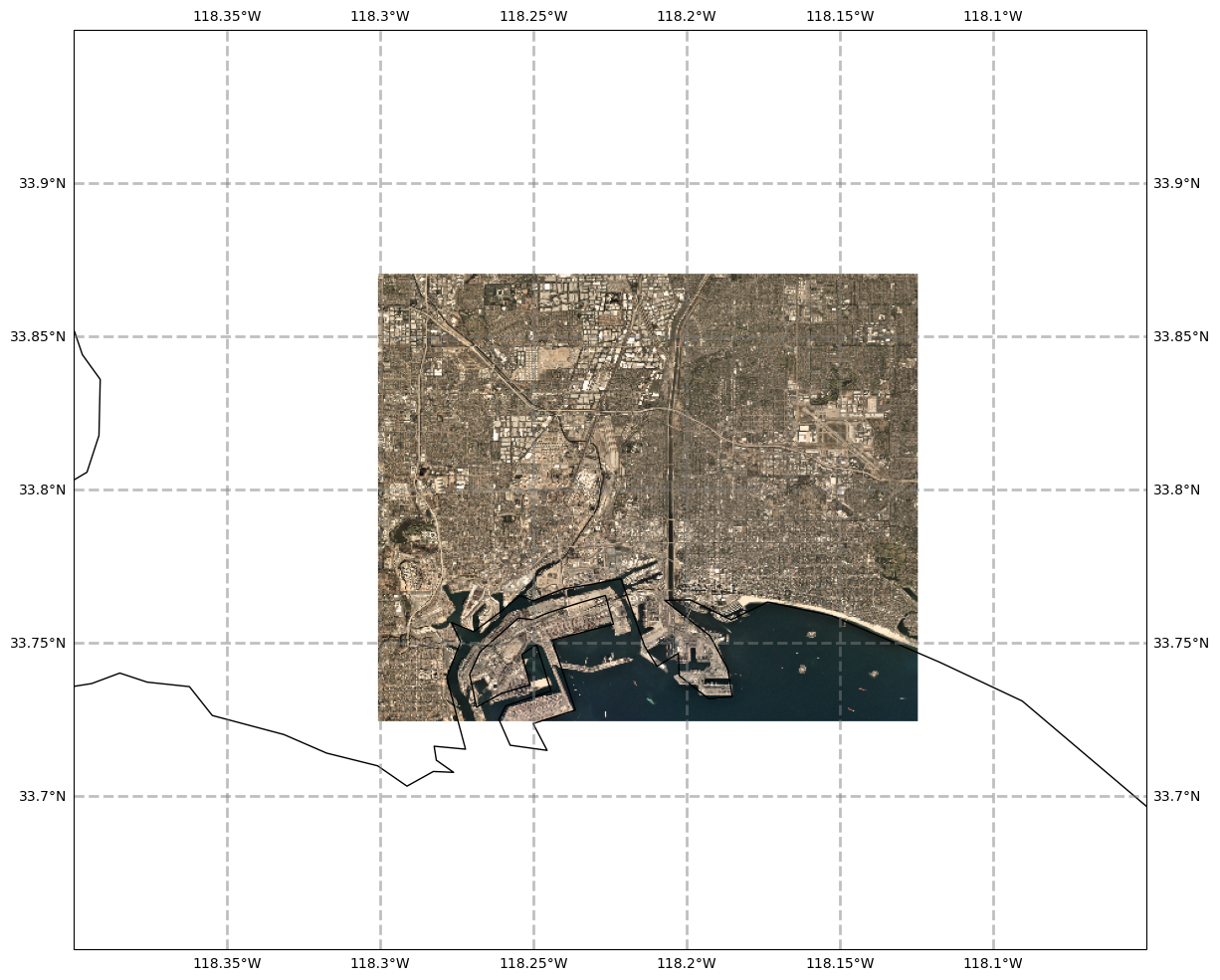
Summary¶
Geo-referenced GeoTIFFs typically consist of three (RGB) or four (RGBA) bands.
Via the
rioxarray
library, these bands make up the data variables when opened as a XarrayDataset
.GeoTIFFs that are optimized for cloud storage are called Cloud-optimized GeoTIFFs (COGs).
Just like any geo-referenced array, COGs can be visualized with Matplotlib and Cartopy.
What’s Next?¶
Raster and vector data are the two core datatypes used in GIS. We’ll next explore vector data, which include shapefiles.
References:¶
https://medium.com/planet-stories/a-handy-introduction-to-cloud-optimized-geotiffs-1f2c9e716ec3
https://medium.com/planet-stories/reading-a-single-tiff-pixel-without-any-tiff-tools-fcbd43d8bd24
https://gis.stackexchange.com/questions/306164/how-to-visualize-multiband-imagery-using-rasterio
https://automating-gis-processes.github.io/CSC/notebooks/L5/plotting-raster.html